The cheapest deposit rates in almost any currencies can be ten and the merely excommunication is actually bitcoin accrual that may’t stay lower than no.02. Almost all a world selling is date plus the representative charges absolutely no money saving deals financing. Read More
Gambling enterprises are working hard to release all favourite games for the ipad, but it is crucial that you understand where to find a secure, reputable web site that may work on the tablet today. You can request profits simply once confirmation, and therefore comes down to filling in the fresh reputation with personal information and you can getting a scanned backup of your own passport. Read More
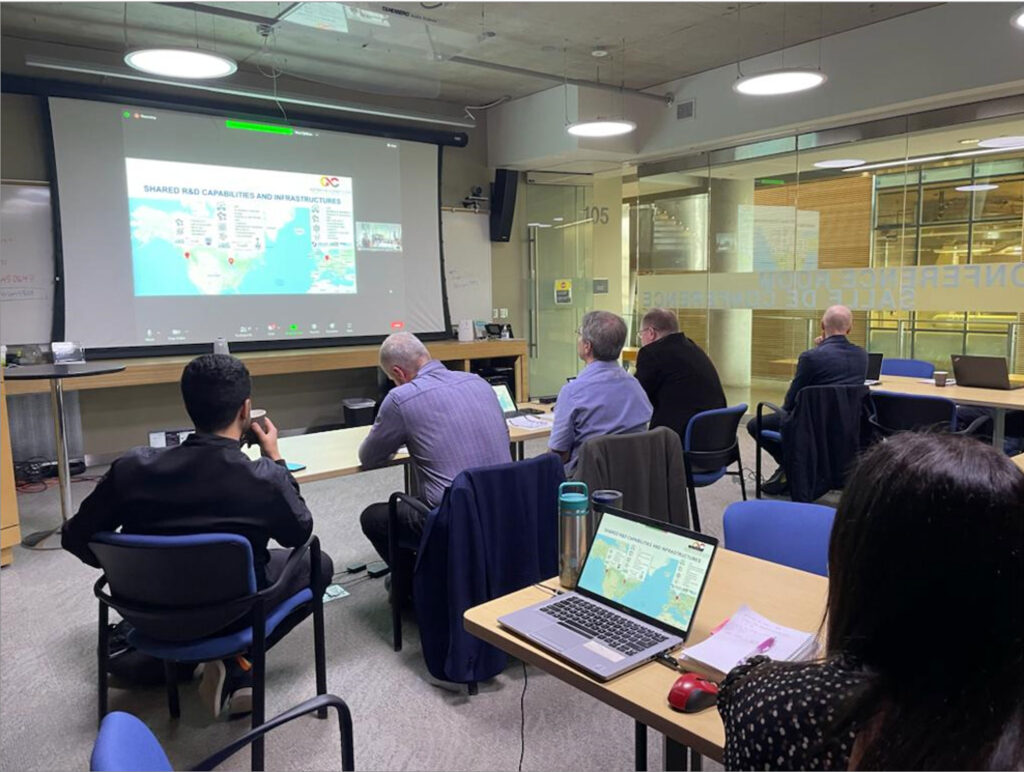
Jülich, 7. Mai 2021 – Mit dem German-Canadian Materials Acceleration Centre (GC-MAC) schaffen das Bundesministerium für Bildung und Forschung (BMBF) und die kanadische Regierung eine gemeinsame Plattform für die rasche Entwicklung klimaneutraler Energietechnologien.
10+ Best WordPress Chatbot Plugins for Your Website in 2024
Even in Image Playground, a new, standalone AI image-generation app, you’re guided to suggestions and limited to select styles. You can’t make photorealistic deepfakes with Apple’s app, in other words. If you want to make your writing more concise or summarize an email, Apple Intelligence can help. If you want to shoot off a quick reply to an email, a suggested reply may be useful here, too. If, however, you want to create an entire bedtime story out of thin air, Apple will offer you the ability to ask ChatGPT for help with that instead.
She acknowledges that any study of AI has a short shelf life — what it failed at today it might grasp tomorrow. Some experts might say that the entire notion of testing machines Chat GPT with methods meant to measure human abilities is anthropomorphizing and wrongheaded. “We find that it’s the worst at causal reasoning — it’s really painfully bad,” Kosoy said.
WPBot requires mysql version 5.6+ for the simple text responses to work. If your server has a version below that, you might see some PHP error or the Simple Text Responses will not work at all. Please request your hosting support to update the mysql version on your server.
Up to 25% of retargeted visitors will respond to your message and turn into customers. Whatever you choose, you will give your site visitors a more personalized experience that answers their questions. First, let’s look into the different types of chatbots so you can choose exactly what you need.
A chatbot is a software tool that uses artificial intelligence to simulate human conversation with website visitors. It’s a useful alternative to live chat, which can be costly and sometimes not very time efficient for some businesses. That’s because a chatbot can carry on multiple conversations at once, wordpress ai chatbot whereas a person trying to answer a dozen questions simultaneously would quickly become overwhelmed. You can use chat flows or a conversational AI, Lyro, for your customer communication. Chat flows are rule-based chatbots that act based on predefined scenarios and use buttons for interactions with users.
These models are trained to understand and respond to user queries in a natural, conversational manner. They’re not just chatbots; they’re intelligent conversational partners that can engage, inform, and assist your visitors in real time. Whether it’s providing detailed answers to complex queries or engaging in casual conversation, these models are equipped to elevate the user experience on your website. You.com is an AI chatbot and search assistant that helps you find information using natural language.
- Implementing a chatbot on your WordPress website can revolutionize your client experience, boost conversion rates, and help your business stand out among competitors.
- The following AI chatbots have been carefully selected based on various factors, including ease of use, features, functionality, pros and cons, and customer reviews.
- With these plugins, you can use AI models like OpenAI’s GPT-3 and GPT-4 to implement your own conversational AI chatbot that’s aware of your site’s content.
- These models are trained to understand and respond to user queries in a natural, conversational manner.
- Give instant answers around the clock and gather more leads based on those positive interactions.
This means the AI is processing the new information, so it can learn to give even better responses. Another way to create a chatbot for your website is to use IBM’s Watson Assistant. This sophisticated AI is available for free through IBM Cloud. While not as straightforward as the previous tool, Watson is very versatile and learns more with every use.
If the shopper denies the offer, Bot will ask for the shopper’s email that will be sent to the shop admin with details about the product and the last offer by the shopper. We help your business grow by connecting you to your customers. Acobot can also interact through voice, meaning customers can reach out to their favorite brands even when their hands are busy. Whether you’re looking for a simple, free option or a lead-generating machine, we’ve got you covered. Propel your customer service to the next level with Tidio’s free courses. Discover how this Shopify store used Tidio to offer better service, recover carts, and boost sales.
I am not getting emails from the ChatBot
Drive customer satisfaction with live chat, ticketing, video calls, and multichannel communication – everything you need for customer service. All messages and live chat conversations are grouped and available in your panel. You can remove them permanently and clear the live chat history manually if you need.
You can foun additiona information about ai customer service and artificial intelligence and NLP. ++ Upgrade to WPBot Pro to power your ChatBot with OpenAI (ChatGPT) fine tuning and GPT assistant features. The company says your Meta AI interactions wouldn’t be used in the future to train its AI. “We have no idea what they use the data for,” said Stefan Baack, a researcher with the Mozilla Foundation who recently analyzed a data repository used by ChatGPT.
It’s an excellent tool for those who prefer a simple and intuitive way to explore the internet and find information. It benefits people who like information presented in a conversational format rather than traditional search result pages. Microsoft Copilot is an AI assistant infused with live web search results from Bing Search.
But she and others argue that to truly understand intelligence and to create it, the learning and reasoning abilities that unfold through childhood can’t be discounted. Current AI is optimized in part with “reinforcement learning from human feedback” — human input on what kind of response is appropriate. While children get that feedback, too, they also have curiosity and an intrinsic drive to explore and seek out information. They figure out how a toy works by shaking it, pushing a button or turning it over — in turn gaining a modicum of control over their environment. To fill this gap, researchers are debating how to program a bit of the child mind into the machine. The most obvious difference is that children don’t learn all of what they know from reading the encyclopedia.
AI chatbot is an advanced chatbot solution specifically designed for websites. It uses AI technology to provide instant and accurate responses to all visitor queries. The chatbot companies don’t tend to detail much about their AI refinement and training processes, including under what circumstances humans might review your chatbot conversations.
Tidio’s chatbot feature is part of its larger customer service suite, which also includes live chat and email integrations. A no-code builder with ready-to-use templates will save you time and money. Instead of spending thousands of dollars on development, you can create chatbots with a drag-and-drop interface.
ChatBot.com
💪 LoryBot is a conversational powerhouse, equipped to handle a wide range of queries, from simple FAQs to more complex questions. Its AI-driven engine is designed to understand and respond effectively to various user inquiries, providing accurate and informative responses. That wraps up our curated list of the best WordPress AI chatbot plugins. In terms of the chatbot functionality, it also does a good job of letting you train your chatbot on your WordPress site’s content and fine-tune everything as much as needed. You also get lots of options to customize how your chatbot interacts with visitors. 🔀 Multiple Assistants, Multiple Roles
Unlock the potential of personalized digital interaction.
Bargain Bot will detect the shopper’s exit intent and ask to offer their own price instead of just leaving. Say goodbye to the old and boring way of offering discounts for the sake of it. Bargaining Bot is the World’s first negotiation bot for WooCommerce. Shoppers are more likely to take advantage of their discount if they have to “work” for it. It makes the shopping experience much more lively and interactive. A personalized welcome message goes a long way to light up one’s day.
Answering common questions is one of the things WordPress chatbots are best at. Fielding the same questions over and over again can massively eat away at your customer service hours. Chatbots don’t get tired of repetitive questions, and they can answer them at any time of day or night. Even better, they’re able to give consistent and instant responses every time with a voice customized to reflect your brand’s unique style. Chatra’s chatbot has robust FAQ functionality, providing instant answers to customers who are too busy to search for answers on their own. Plus with mobile access on iOS and Android devices, agents can stay close at hand no matter where they are in case the conversation needs human intervention.
- A personalized welcome message goes a long way to light up one’s day.
- Jasper AI is a boon for content creators looking for a smart, efficient way to produce SEO-optimized content.
- The company says your Meta AI interactions wouldn’t be used in the future to train its AI.
- It’s all part of an effort to say that, this time, when the shareholders vote to approve his monster $56 billion compensation package, they were fully informed.
- The AI Playground offers a range of AI tools, including translation, correction, SEO, suggestions, and others.
- It offers many of the same features but has chosen to specialize in a few areas where they fall short.
Companies already committed to HubSpot’s CRM will find their basic live chat needs to be met, although it lacks advanced conversational AI capabilities. This platform offers a two-in-one solution for those seeking a CRM and a chatbot. Lyro AI by Tidio uses your content and data to make chats as smooth as possible. They can take FAQs and give them to your visitors in a way that matches the flow of the conversation.
It is also important to check your usage on the OpenAI website for accurate information. Under privacy laws in some parts of the world, including the European Union, Meta must offer “objection” options for the company’s use of personal data. The objection forms aren’t an option for people in the United States. Read more from Google here, including options to automatically delete your chat conversations with Gemini. She’s heard of friends copying group chat messages into a chatbot to summarize what they missed while on vacation. Mireshghallah was part of a team that analyzed publicly available ChatGPT conversations and found a significant percentage of the chats were sex-related.
Divi Page Builder Plugin
When Schulz pushed back, reminding ChatGPT that both partners had to win to get a prize, it doubled down on its answer. Children, on the other hand, are thought by many developmental psychologists to have some core set of cognitive abilities. What exactly they are remains a matter of scientific investigation, but they seem to allow kids to get a lot of new knowledge out of a little input.
Monitor the performance of your team, Lyro AI Chatbot, and Flows. You can schedule your operating hours or show the widget only when you are online. You can also set up automatic responses to be sent on specific days of the week. As you can see, there’s nothing too complex about this operation, is there? When you’ve finished customizing the settings, there’s no need to save.
It works smoothly with ChatBot.com’s sister brands—LiveChat.com and HelpDesk.com—providing a whole enterprise support framework. For businesses on the cusp of significant growth, the ChatBot.com suite is a worthy choice. Next up, DocsBot AI is another sophisticated and trainable AI solution that transforms traditional documentation into chatbots.
Another popular option is combining an LLM chatbot with a live chat fallback option. If you are interested to know about the best AI chatbots for customer support, click here. Using a chatbot for your WordPress websites has many advantages. But choosing the right chatbot is an important step towards it.
This will help you stay organized and measure the results of your bot down the line. These will help you keep an eye on the chatbot’s performance and improve it quickly. You’ll be able to see the areas in which the bot needs improvements and which ones are performing well. Let’s check out the benefits of a website chatbot for WordPress in more detail. Expanding the lines of what is possible and what we can do with technology, Open AI can be used for a variety of tasks. These include having a conversation with the user, creating long pieces of content, writing code, and much more.
Can I customize the appearance of the chatbot?
In some cases, researchers said, Copilot combined different polling numbers into one answer, creating something totally incorrect out of initially accurate data. The chatbot would also link to accurate sources online, but then screw up its summary of the provided information. Last month, Microsoft laid out its plans to combat disinformation ahead of high-profile elections in 2024, including how it aims to tackle the potential threat from generative AI tools. These issues regarding election misinformation also do not appear to have been addressed on a global scale, as the chatbot’s responses to WIRED’s 2024 US election queries show. Jasper is another AI chatbot and writing platform, but this one is built for business professionals and writing teams. While there is much more to Jasper than its AI chatbot, it’s a tool worth using.
Tidio is a free WordPress chatbot plugin that has over a dozen templates for recovering abandoned carts, offering discounts and promotions, and collecting leads. Or, for those who prefer to create their own conversations, Tidio has a drag-and-drop visual editor that allows users to create conversations from scratch. Trigger conversations by defined actions, or customize triggers to reach out at the right moment. If you’re new in business or a freelancer, you’re likely seeking an affordable, or even free, WordPress chat assistant platform that provides basic features. Since you might not receive an overwhelming number of inquiries, a heavy-duty enterprise system isn’t necessary.
7 Best Chatbots Of 2024 – Forbes Advisor – Forbes
7 Best Chatbots Of 2024 – Forbes Advisor.
Posted: Mon, 01 Apr 2024 07:00:00 GMT [source]
It is a visual, drag and drop form builder that is easy to use and very flexible. Supports conditional logic and use of variables to build all types of forms or just menu driven conversations with if else logic. Conversations or forms can be eMailed to you and saved in the database. ChatBot is perfect for companies seeking a comprehensive digital assistant for sales and customer care.
This feature enhances the user experience and provides a unique way to engage with your audience. By integrating the IBM Watson-powered chatbot plugin into your WordPress site, you can revolutionize customer support and enhance their experience. One key thing to remember before beginning your chatbot journey is to do your research beforehand, to ensure you know what features are best suited for your business needs. You should also take your team’s IT capabilities into account, since some platforms will have a much steeper learning curve than others.
Does LoryBot require technical expertise to set up?
Copilot represents the leading brand of Microsoft’s AI products, but you have probably heard of Bing AI (or Bing Chat), which uses the same base technologies. Copilot extends to multiple surfaces and is usable on its own landing page, in Bing search results, and increasingly in other Microsoft products and operating systems. Bing is an exciting chatbot because of its close ties with ChatGPT.
With quick answers and helpful guidance, LoryBot ensures your website visitors feel supported and satisfied. However, it doesn’t support any chatbot functionality in the free version, so it’s probably not the best choice if you’re on a tight budget. The developer also has a guide on how to train your own AI model, which makes it easy to get started. It’s one of the better documented WordPress AI chatbot plugins that we found, which is great if this is your first step into the space. Whether your visitors are looking for product information, need support, or have general inquiries, the AI chatbot plugin for the website, known as Robofy, has got it covered.
Landbot.io chatbots also include surveys designed to keep customers engaged so they don’t get bored with long drawn-out forms and questionnaires. For employers looking to simplify the onboarding process, Landbot.io can even be configured to help guide new hires through learning the ropes. Just install the plugin with a click, then choose from over 100 templates or build a conversation from scratch using the drag ‘n drop builder.
From 24/7 customer support responses to sales information and marketing, you’ll likely be able to find a way chatbots can work for you. A separate FaceBook Messenger ChatBot addon is available that extends the WPBot’s functionality so the ChatBot can chat with your users on your Facebook Page & Facebook Messenger. AI Engine is one of the best WordPress AI chatbot plugins in the WordPress.org directory – and among the most popular. It’s a full-service solution for integrating AI into your site, including creating content with AI, generating images, and – you guessed it – creating an AI chatbot. In this post, we’ve collected our picks for the four best WordPress AI chatbot plugins.
Display a translated version of the widget based on the customer’s location. The second step required to take full advantage of the plugin’s features is to connect it to an artificial https://chat.openai.com/ intelligence service. For this, the plugin’s author recommends OpenAI, the parent company of the famous ChatGPT. And by the way, 70% of customers find the experience positive.
Transfer high-intent leads to your sales reps in real time to shorten the sales cycle. The FAQ module has priority over AI Assist, giving you power over the collected questions and answers used as bot responses. Here’s a look at all our featured chatbots to see how they compare in pricing. The chat interface is simple and makes it easy to talk to different characters.
📊 Direct Traffic with Customizable Buttons
Guide your visitors where you want them. Customizable buttons can link directly to specific pages, forms, or contact information, facilitating smoother navigation and enhanced user engagement. The WordPress AI Chatbot can handle a wide range of inquiries, including product information, support assistance, general inquiries, and more. I am thrilled about the endless opportunities that AI brings.
You have to admit that there are many advantages to using a chatbot, though there are certain disadvantages as well. In contrast, an intelligent chatbot doesn’t just use keywords. It analyzes the meaning of the sentence and learns from past interactions with your visitors, in order to respond more precisely. Chatbots are becoming increasingly widespread in a variety of fields, including customer service, marketing, task management, etc. Some of them require upgrading the plugin, but there’s enough functionality in the free version to get you started. Thanks to the In-Chat Search they will be able to search their answer in there without bugging your customer support team.
Initially restricted to Microsoft’s Edge browser, that chatbot has since been made available on other browsers and on smartphones. Anyone searching on Bing can now receive a conversational response that draws from various sources rather than just a static list of links. Enhance your AI chatbot with new features, workflows, and automations through plug-and-play integrations. Reach out to visitors proactively using personalized chatbot greetings. Help your business grow with the best chatbot app by combining automated AI answers with dedicated flows. ChatBot scans your website, help center, or other designated resource to provide quick and accurate AI-generated answers to customer questions.
Symbolic AI vs Machine Learning in Natural Language Processing
However, neural networks fell out of favor in 1969 after AI pioneers Marvin Minsky and Seymour Papert published a paper criticizing their ability to learn and solve complex problems. Popular categories of ANNs include convolutional neural networks (CNNs), recurrent neural networks (RNNs) and transformers. CNNs are good at processing information in parallel, such as the meaning of pixels in an image. New GenAI techniques often use transformer-based neural networks that automate data prep work in training AI systems such as ChatGPT and Google Gemini.
One of their projects involves technology that could be used for self-driving cars. Consequently, learning to drive safely requires enormous amounts of training data, and the AI cannot be trained out in the real world. Such causal and counterfactual reasoning about things that are changing with time is extremely difficult for today’s deep neural networks, which mainly excel at discovering static patterns in data, Kohli says. The researchers broke the problem into smaller chunks familiar from symbolic AI. In essence, they had to first look at an image and characterize the 3-D shapes and their properties, and generate a knowledge base.
Through symbolic representations of grammar, syntax, and semantic rules, AI models can interpret and produce meaningful language constructs, laying the groundwork for language translation, sentiment analysis, and chatbot interfaces. For other AI programming languages see this list of programming languages for artificial intelligence. Currently, Python, a multi-paradigm programming language, is the most popular programming language, partly due to its extensive package library that supports data science, natural language processing, and deep learning. Python includes a read-eval-print loop, functional elements such as higher-order functions, and object-oriented programming that includes metaclasses. Symbolic AI, also referred to as “good old fashioned AI” (GOFAI), employs symbolic representations and logic-based rules to perform tasks that require human-like intelligence.
What is the difference between statistical AI and symbolic AI?
While symbolic AI accomplishes tasks through knowledge encoding and reasoning principles, statistical AI depends on data analysis and prediction to make judgments. Researchers often mix the two methods in order to build more robust AI systems, as each has its advantages and disadvantages.
This section outlines a comprehensive roadmap for developing Symbolic AI systems, addressing practical considerations and best practices throughout the process. One of the critical limitations of Symbolic AI, highlighted by the GHM source, is its inability to learn and adapt by itself. The grandfather of AI, Thomas Hobbes said — Thinking is manipulation of symbols and Reasoning is computation. These potential applications demonstrate the ongoing relevance and potential of Symbolic AI in the future of AI research and development.
Hatchlings shown two red spheres at birth will later show a preference for two spheres of the same color, even if they are blue, over two spheres that are each a different color. Somehow, the ducklings pick up and imprint on the idea of similarity, in this case the color of the objects. So not only has symbolic AI the most mature and frugal, it’s also the most transparent, and therefore accountable. As pressure mounts on GAI companies to explain where their apps’ answers come from, symbolic AI will never have that problem. This impact is further reduced by choosing a cloud provider with data centers in France, as Golem.ai does with Scaleway. As carbon intensity (the quantity of CO2 generated by kWh produced) is nearly 12 times lower in France than in the US, for example, the energy needed for AI computing produces considerably less emissions.
Neuro-symbolic AI for scene understanding
Qualitative simulation, such as Benjamin Kuipers’s QSIM,[88] approximates human reasoning about naive physics, such as what happens when we heat a liquid in a pot on the stove. We expect it to heat and possibly boil over, even though we may not know its temperature, its boiling point, or other details, such as atmospheric pressure. A more flexible kind of problem-solving occurs when reasoning about what to do next occurs, rather than simply choosing one of the available actions. This kind of meta-level reasoning is used in Soar and in the BB1 blackboard architecture.
What is symbolic NLP?
The symbolic approach applied to NLP
With this approach, also called “deterministic”, the idea is to teach the machine how to understand languages in the same way as we, humans, have learned how to read and how to write.
In these fields, Symbolic AI has had limited success and by and large has left the field to neural network architectures (discussed in a later chapter) which are more suitable for such tasks. In sections to follow we will elaborate on important sub-areas of Symbolic AI as well as difficulties encountered by this approach. For example, AI models might benefit from combining more structural information across various levels of abstraction, such as transforming a raw invoice document into information about purchasers, products and payment terms. An internet of things stream could similarly benefit from translating raw time-series data into relevant events, performance analysis data, or wear and tear. Future innovations will require exploring and finding better ways to represent all of these to improve their use by symbolic and neural network algorithms.
Need for Neuro Symbolic AI
Symbolic AI has been criticized as disembodied, liable to the qualification problem, and poor in handling the perceptual problems where deep learning excels. In Symbolic AI, knowledge is explicitly encoded in the form of symbols, rules, and relationships. These symbols can represent objects, concepts, or situations, and the rules define how these symbols can be manipulated or combined to derive new knowledge or make inferences.
For example, DeepMind’s AlphaGo used symbolic techniques to improve the representation of game layouts, process them with neural networks and then analyze the results with symbolic techniques. Other potential use cases of deeper neuro-symbolic integration include improving explainability, labeling data, reducing hallucinations and discerning cause-and-effect relationships. Psychologist Daniel Kahneman suggested that neural networks and symbolic approaches correspond to System 1 and System 2 modes of thinking and reasoning. System 1 thinking, as exemplified in neural AI, is better suited for making quick judgments, such as identifying a cat in an image. System 2 analysis, exemplified in symbolic AI, involves slower reasoning processes, such as reasoning about what a cat might be doing and how it relates to other things in the scene. Symbolic AI, a branch of artificial intelligence, excels at handling complex problems that are challenging for conventional AI methods.
For much of the AI era, symbolic approaches held the upper hand in adding value through apps including expert systems, fraud detection and argument mining. But innovations in deep learning and the infrastructure for training large language models (LLMs) have shifted the focus toward neural networks. One such project is the Neuro-Symbolic Concept Learner (NSCL), a hybrid AI system developed by the MIT-IBM Watson AI Lab.
What is the scope of symbolic AI?
In natural language processing, Symbolic AI is used to represent and manipulate linguistic symbols, enabling machines to interpret and generate human language. This facilitates tasks such as language translation, semantic analysis, and conversational understanding.
But they require a huge amount of effort by domain experts and software engineers and only work in very narrow use cases. As soon as you generalize the problem, there will be an explosion of new rules to add (remember the cat detection problem?), which will require more human labor. One of the main stumbling blocks of symbolic AI, or GOFAI, was the difficulty of revising beliefs once they were encoded in a rules engine. Expert systems are monotonic; that is, the more rules you add, the more knowledge is encoded in the system, but additional rules can’t undo old knowledge.
Reasons Conversational AI is a Must-Have for Businesses This Holiday
Like Inbenta’s, “our technology is frugal in energy and data, it learns autonomously, and can explain its decisions”, affirms AnotherBrain on its website. And given the startup’s founder, Bruno Maisonnier, previously founded Aldebaran Robotics (creators of the NAO and Pepper robots), AnotherBrain is unlikely to be a flash in the pan. As such, Golem.ai applies linguistics and neurolinguistics to a given problem, rather than statistics. Their algorithm includes almost every known language, enabling the company to analyze large amounts of text. Notably because unlike GAI, which consumes considerable amounts of energy during its training stage, symbolic AI doesn’t need to be trained.
New deep learning approaches based on Transformer models have now eclipsed these earlier symbolic AI approaches and attained state-of-the-art performance in natural language processing. However, Transformer models are opaque and do not yet produce human-interpretable semantic representations for sentences and documents. Instead, they produce task-specific vectors where the meaning of the vector components is opaque. Symbolic AI algorithms are designed to deal with the kind of problems that require human-like reasoning, such as planning, natural language processing, and knowledge representation. Better yet, the hybrid needed only about 10 percent of the training data required by solutions based purely on deep neural networks. When a deep net is being trained to solve a problem, it’s effectively searching through a vast space of potential solutions to find the correct one.
Neural Networks excel in learning from data, handling ambiguity, and flexibility, while Symbolic AI offers greater explainability and functions effectively with less data. Rule-Based AI, a cornerstone of Symbolic AI, involves creating AI systems that apply predefined rules. This concept is fundamental in AI Research Labs and universities, contributing to significant Development Milestones in AI. RAAPID’s retrospective and prospective solution is powered by Neuro-symbolic AI to revolutionize chart coding, reviewing, auditing, and clinical decision support. Our Neuro-Symbolic AI solutions are meticulously curated from over 10 million charts, encompassing over 4 million clinical entities and over 50 million relationships.
While efficient for tasks with clear rules, it often struggles in areas requiring adaptability and learning from vast data. The strengths of subsymbolic AI lie in its ability to handle complex, unstructured, and noisy data, such as images, speech, and natural language. This approach has been particularly successful in tasks like computer vision, speech recognition, and language understanding.
This aspect also saves time compared with GAI, as without the need for training, models can be up and running in minutes. In response to these challenges, recent advancements in Symbolic AI have focused on integrating machine learning techniques to automate knowledge acquisition and enhance the system’s ability to learn and adapt. Symbolic AI holds a special place in the quest for AI that not only performs complex tasks but also https://chat.openai.com/ provides clear insights into its decision-making processes. This quality is indispensable in applications where understanding the rationale behind AI decisions is paramount. A certain set of structural rules are innate to humans, independent of sensory experience. With more linguistic stimuli received in the course of psychological development, children then adopt specific syntactic rules that conform to Universal grammar.
Challenges of Knowledge Acquisition and Maintenance
This approach involves creating explicit maps of the world and associating symbols with different objects or concepts, allowing for the manipulation and interpretation of these symbols according to predefined rules. Neuro symbolic AI is a topic that combines ideas from deep neural networks with symbolic reasoning and learning to overcome several significant technical hurdles such as explainability, modularity, verification, and the enforcement of constraints. While neuro symbolic ideas date back to the early 2000’s, there have been significant advances in the last five years. Symbolic AI algorithms are used in a variety of applications, including natural language processing, knowledge representation, and planning. We see Neuro-symbolic AI as a pathway to achieve artificial general intelligence. By augmenting and combining the strengths of statistical AI, like machine learning, with the capabilities of human-like symbolic knowledge and reasoning, we’re aiming to create a revolution in AI, rather than an evolution.
“It’s one of the most exciting areas in today’s machine learning,” says Brenden Lake, a computer and cognitive scientist at New York University. Symbolic AI, a fascinating subfield of artificial intelligence, stands out by focusing on the manipulation and processing of symbols and concepts rather than numerical data. This unique approach allows for the representation of objects and ideas in a way that’s remarkably similar to human thought processes. There have been several efforts to create complicated symbolic AI systems that encompass the multitudes of rules of certain domains. Called expert systems, these symbolic AI models use hardcoded knowledge and rules to tackle complicated tasks such as medical diagnosis.
Artificial Experientialism (AE), rooted in the interplay between depth and breadth, provides a novel lens through which we can decipher the essence of artificial experience. Unlike humans, AI does not possess a biological or emotional consciousness; instead, its ‘experience’ can be viewed as a product of data processing and pattern recognition (Searle, 1980). The difficulties encountered by symbolic AI have, however, been deep, possibly unresolvable ones. One difficult problem encountered by symbolic AI pioneers came to be known as the common sense knowledge problem.
Agents and multi-agent systems
Thus contrary to pre-existing cartesian philosophy he maintained that we are born without innate ideas and knowledge is instead determined only by experience derived by a sensed perception. Children can be symbol manipulation and do addition/subtraction, but they don’t really understand what they are doing. However, this also required much manual effort from experts tasked with deciphering the chain of thought processes that connect various symptoms to diseases or purchasing patterns to fraud. This downside is not a big issue with deciphering the meaning of children’s stories or linking common knowledge, but it becomes more expensive with specialized knowledge. For example, AI developers created many rule systems to characterize the rules people commonly use to make sense of the world. This resulted in AI systems that could help translate a particular symptom into a relevant diagnosis or identify fraud.
Again, this stands in contrast to neural nets, which can link symbols to vectorized representations of the data, which are in turn just translations of raw sensory data. So the main challenge, when we think about GOFAI and neural nets, is how to ground symbols, or relate them to other forms of meaning that would allow computers to map the changing raw sensations of the world to symbols and then reason about them. Chat GPT The neuro-symbolic model, NSCL, excels in this task, outperforming traditional models, emphasizing the potential of Neuro-Symbolic AI in understanding and reasoning about visual data. Notably, models trained on the CLEVRER dataset, which encompasses 10,000 videos, have outperformed their traditional counterparts in VQA tasks, indicating a bright future for Neuro-Symbolic approaches in visual reasoning.
With its combination of deep learning and logical inference, neuro-symbolic AI has the potential to revolutionize the way we interact with and understand AI systems. Due to the shortcomings of these two methods, they have been combined to create neuro-symbolic AI, which is more effective than each alone. According to researchers, deep learning is expected to benefit from integrating domain knowledge and common sense reasoning provided by symbolic AI systems. For instance, a neuro-symbolic system would employ symbolic AI’s logic to grasp a shape better while detecting it and a neural network’s pattern recognition ability to identify items. First of all, every deep neural net trained by supervised learning combines deep learning and symbolic manipulation, at least in a rudimentary sense.
All of this is encoded as a symbolic program in a programming language a computer can understand. In ML, knowledge is often represented in a high-dimensional space, which requires a lot of computing power to process and manipulate. In contrast, symbolic AI uses more efficient algorithms and techniques, such as rule-based systems and logic programming, which require less computing power.
Neuro-Symbolic AI Could Redefine Legal Practices – Forbes
Neuro-Symbolic AI Could Redefine Legal Practices.
Posted: Wed, 15 May 2024 07:00:00 GMT [source]
Despite its strengths, Symbolic AI faces challenges, such as the difficulty in encoding all-encompassing knowledge and rules, and the limitations in handling unstructured data, unlike AI models based on Neural Networks and Machine Learning. Symbolic AI’s logic-based approach contrasts with Neural Networks, which are pivotal in Deep Learning and Machine Learning. Neural Networks learn from data patterns, evolving through AI Research and applications.
Say you have a picture of your cat and want to create a program that can detect images that contain your cat. You create a rule-based program that takes new images as inputs, compares the pixels to the original cat image, and responds by saying whether your cat is in those images. Symbolic artificial intelligence showed early progress at the dawn of AI and computing. You can easily visualize the logic of rule-based programs, communicate them, and troubleshoot them. Using symbolic AI, everything is visible, understandable and explainable, leading to what is called a ‘transparent box’ as opposed to the ‘black box’ created by machine learning.
Other non-monotonic logics provided truth maintenance systems that revised beliefs leading to contradictions. Limitations were discovered in using simple first-order logic to reason about dynamic domains. Problems were discovered both with regards to enumerating the preconditions for an action to succeed and in providing axioms for what did not change after an action was performed. The General Problem Solver (GPS) cast planning as problem-solving used means-ends analysis to create plans. Graphplan takes a least-commitment approach to planning, rather than sequentially choosing actions from an initial state, working forwards, or a goal state if working backwards. Satplan is an approach to planning where a planning problem is reduced to a Boolean satisfiability problem.
This simple symbolic intervention drastically reduces the amount of data needed to train the AI by excluding certain choices from the get-go. “If the agent doesn’t need to encounter a bunch of bad states, then it needs less data,” says Fulton. While the project still isn’t ready for use outside the lab, Cox envisions a future in which cars with neurosymbolic AI could learn out in the real world, with the symbolic component acting as a bulwark against bad driving.
However, interest in all AI faded in the late 1980s as AI hype failed to translate into meaningful business value. Symbolic AI emerged again in the mid-1990s with innovations in machine learning techniques that could automate the training of symbolic systems, such as hidden Markov models, Bayesian networks, fuzzy logic and decision tree learning. Our model builds an object-based scene representation and translates sentences into executable, symbolic programs.
If I tell you that I saw a cat up in a tree, your mind will quickly conjure an image. The effectiveness of symbolic AI is also contingent on the quality of human input. The systems depend on accurate and comprehensive knowledge; any deficiencies in this data can lead to subpar AI performance.
By the end of this exploration, readers will gain a profound understanding of the importance and impact of symbolic AI in the domain of artificial intelligence. Knowledge-based systems have an explicit knowledge base, typically of rules, to enhance reusability across domains by separating procedural code and domain knowledge. A separate inference engine processes rules and adds, deletes, or modifies a knowledge store. Semantic networks, what is symbolic ai conceptual graphs, frames, and logic are all approaches to modeling knowledge such as domain knowledge, problem-solving knowledge, and the semantic meaning of language. DOLCE is an example of an upper ontology that can be used for any domain while WordNet is a lexical resource that can also be viewed as an ontology. YAGO incorporates WordNet as part of its ontology, to align facts extracted from Wikipedia with WordNet synsets.
Planning is used in a variety of applications, including robotics and automated planning. Symbolic AI algorithms are based on the manipulation of symbols and their relationships to each other. Knowledge graph embedding (KGE) is a machine learning task of learning a latent, continuous vector space representation of the nodes and edges in a knowledge graph (KG) that preserves their semantic meaning. This learned embedding representation of prior knowledge can be applied to and benefit a wide variety of neuro-symbolic AI tasks.
What is the opposite of symbolic AI?
Non-symbolic AI systems do not manipulate a symbolic representation to find solutions to problems. Instead, they perform calculations according to some principles that have demonstrated to be able to solve problems.
By combining symbolic and neural reasoning in a single architecture, LNNs can leverage the strengths of both methods to perform a wider range of tasks than either method alone. For example, an LNN can use its neural component to process perceptual input and its symbolic component to perform logical inference and planning based on a structured knowledge base. When considering how people think and reason, it becomes clear that symbols are a crucial component of communication, which contributes to their intelligence. Researchers tried to simulate symbols into robots to make them operate similarly to humans. This rule-based symbolic Artifical General Intelligence (AI) required the explicit integration of human knowledge and behavioural guidelines into computer programs. Additionally, it increased the cost of systems and reduced their accuracy as more rules were added.
Below is a quick overview of approaches to knowledge representation and automated reasoning. This article was written to answer the question, “what is symbolic artificial intelligence.” Looking to enhance your understanding of the world of AI? So, to verify Elvis Presley’s birthplace, specifically whether he was born in England refer the above diagram , the system initially converts the question into a generic logical form by translating it into an Abstract Meaning Representation (AMR). Each AMR encapsulates the meaning of the question using terminology independent of the knowledge graph, a crucial feature enabling the technology’s application across various tasks and knowledge bases. Symbolic AI is able to deal with more complex problems, and can often find solutions that are more elegant than those found by traditional AI algorithms.
In contrast to the US, in Europe the key AI programming language during that same period was Prolog. Prolog provided a built-in store of facts and clauses that could be queried by a read-eval-print loop. The store could act as a knowledge base and the clauses could act as rules or a restricted form of logic. At the height of the AI boom, companies such as Symbolics, LMI, and Texas Instruments were selling LISP machines specifically targeted to accelerate the development of AI applications and research. In addition, several artificial intelligence companies, such as Teknowledge and Inference Corporation, were selling expert system shells, training, and consulting to corporations.
Symbolic AI algorithms are able to solve problems that are too difficult for traditional AI algorithms. Symbolic AI and Neural Networks are distinct approaches to artificial intelligence, each with its strengths and weaknesses. Always consider the specific context and application when implementing these insights. In practice, the effectiveness of Symbolic AI integration with legacy systems would depend on the specific industry, the legacy system in question, and the challenges being addressed. If you’re aiming for a specific application or case study, deeper research and consultation with experts in the field might be necessary. For industries where stakes are high, like healthcare or finance, understanding and trusting the system’s decision-making process is crucial.
Constraint logic programming can be used to solve scheduling problems, for example with constraint handling rules (CHR). The logic clauses that describe programs are directly interpreted to run the programs specified. No explicit series of actions is required, as is the case with imperative programming languages. The key AI programming language in the US during the last symbolic AI boom period was LISP. LISP is the second oldest programming language after FORTRAN and was created in 1958 by John McCarthy. LISP provided the first read-eval-print loop to support rapid program development.
It’s more than just advanced intelligence; it’s AI designed to mirror human understanding. As we leverage the full range of AI strategies, we’re not merely progressing—we’re reshaping the AI landscape. Symbolic AI bridges this gap, allowing legacy systems to scale and work with modern data streams, incorporating the strengths of neural models where needed. By combining learning and reasoning, these systems could potentially understand and interact with the world in a way that is much closer to how humans do.
By seamlessly integrating a Clinical Knowledge Graph with Neuro-Symbolic AI capabilities, RAAPID ensures a comprehensive understanding of intricate clinical data, facilitating precise risk assessment and decision support. Our solution, meticulously crafted from extensive clinical records, embodies a groundbreaking advancement in healthcare analytics. In the context of autonomous driving, knowledge completion with KGEs can be used to predict entities in driving scenes that may have been missed by purely data-driven techniques. For example, consider the scenario of an autonomous vehicle driving through a residential neighborhood on a Saturday afternoon. This prediction task requires knowledge of the scene that is out of scope for traditional computer vision techniques.
- The researchers broke the problem into smaller chunks familiar from symbolic AI.
- However, this also required much manual effort from experts tasked with deciphering the chain of thought processes that connect various symptoms to diseases or purchasing patterns to fraud.
- Samuel’s Checker Program[1952] — Arthur Samuel’s goal was to explore to make a computer learn.
- They can learn to perform tasks such as image recognition and natural language processing with high accuracy.
- We’ve been working for decades to gather the data and computing power necessary to realize that goal, but now it is available.
Give the Composer specific instructions, notes, and references from your research and generate quality drafts, outlines, and summaries for your story. RAAPID’s retrospective and prospective risk adjustment solution uses a Clinical Knowledge Graph, a dataset that structures diverse clinical data into a comprehensive, interconnected entity. AE fills this void, offering a comprehensive framework that encapsulates the AI experience. The philosophy of Artificial Experientialism (AE) is fundamentally rooted in understanding this dichotomy.
Additionally, it would utilize a symbolic system to reason about these recognized objects and make decisions aligned with traffic rules. This amalgamation enables the self-driving car to interact with its surroundings in a manner akin to human cognition, comprehending the context and making reasoned judgments. Upon delving into human cognition and reasoning, it’s evident that symbols play a pivotal role in concept understanding and decision-making, thereby enhancing intelligence. Researchers endeavored to emulate this symbol-centric aspect in robots to align their operations closely with human capabilities. This entailed incorporating explicit human knowledge and behavioral guidelines into computer programs, forming the basis of rule-based symbolic AI. However, this approach heightened system costs and diminished accuracy with the addition of more rules.
In Symbolic AI, Knowledge Representation is essential for storing and manipulating information. It is crucial in areas like AI History and development, where representing complex AI Research and AI Applications accurately is vital. At the heart of Symbolic AI lie key concepts such as Logic Programming, Knowledge Representation, and Rule-Based AI.
Q&A: Can Neuro-Symbolic AI Solve AI’s Weaknesses? – TDWI
Q&A: Can Neuro-Symbolic AI Solve AI’s Weaknesses?.
Posted: Mon, 08 Apr 2024 07:00:00 GMT [source]
By enhancing and merging the strengths of statistical AI, such as machine learning, with human-like symbolic knowledge capabilities and reasoning, they aim to spark a revolution in the field of AI. By integrating these methodologies, neuro-symbolic AI aims to develop systems with the dual ability to learn from data and engage in reasoning akin to humans. You can foun additiona information about ai customer service and artificial intelligence and NLP. Samuel’s Checker Program[1952] — Arthur Samuel’s goal was to explore to make a computer learn. The program improved as it played more and more games and ultimately defeated its own creator. This lead towards the connectionist paradigm of AI, also called non-symbolic AI which gave rise to learning and neural network-based approaches to solve AI. During the first AI summer, many people thought that machine intelligence could be achieved in just a few years.
Its history was also influenced by Carl Hewitt’s PLANNER, an assertional database with pattern-directed invocation of methods. For more detail see the section on the origins of Prolog in the PLANNER article. The future includes integrating Symbolic AI with Machine Learning, enhancing AI algorithms and applications, a key area in AI Research and Development Milestones in AI.
He also has full transparency on how to fine-tune the engine when it doesn’t work properly as he’s been able to understand why a specific decision has been made and has the tools to fix it. When deep learning reemerged in 2012, it was with a kind of take-no-prisoners attitude that has characterized most of the last decade. He gave a talk at an AI workshop at Stanford comparing symbols to aether, one of science’s greatest mistakes.
Symbolic AI offers clear advantages, including its ability to handle complex logic systems and provide explainable AI decisions. Neural Networks, compared to Symbolic AI, excel in handling ambiguous data, a key area in AI Research and applications involving complex datasets. Domain2– The structured reasoning and interpretive capabilities characteristic of symbolic AI.
Not everyone agrees that neurosymbolic AI is the best way to more powerful artificial intelligence. Serre, of Brown, thinks this hybrid approach will be hard pressed to come close to the sophistication of abstract human reasoning. Our minds create abstract symbolic representations of objects such as spheres and cubes, for example, and do all kinds of visual and nonvisual reasoning using those symbols. We do this using our biological neural networks, apparently with no dedicated symbolic component in sight.
It follows that neuro-symbolic AI combines neural/sub-symbolic methods with knowledge/symbolic methods to improve scalability, efficiency, and explainability. If the knowledge is incomplete or inaccurate, the results of the AI system will be as well. The development of neuro-symbolic AI is still in its early stages, and much work must be done to realize its potential fully. However, the progress made so far and the promising results of current research make it clear that neuro-symbolic AI has the potential to play a major role in shaping the future of AI. These are just a few examples, and the potential applications of neuro-symbolic AI are constantly expanding as the field of AI continues to evolve. Symbolic AI can handle these tasks optimally, where purely connectionist approaches might falter.
What is beyond limits symbolic AI?
Beyond Limits' Hybrid AI platform combines game-changing Symbolic AI reasoner technology with Numeric AI (Machine Learning, Neural networks and Deep Learning) models and Generative AI to transform knowledge and operational data into intelligent inferences, decisioning workflows and actionable recommendations for …
A Guide to Using AI Chatbots in eCommerce
Enter Giosg AI enables you to build knowledge bases with your chat logs and live conversation history. English is a language with a very wide reach, no doubt; however, some customers might prefer communication in a native language. To breach the language barrier, an eCommerce AI chatbot must possess multi-language support for the elementary kind of requests at least. Businesses are no exception to this trend; as businesses increasingly demand and prefer voice as their primary form of communication, it makes sense to take advantage of the numerous AI use cases in e-commerce. It’s not just for the customer; it also enables your firm to decrease operating expenses and increase operations significantly. With Conversational AI, the user can access all communication channels 24 hours a day.
The values of Cronbach’s Alpha are well above the threshold value of 0.70, which confirms the presence of scale reliability (Portney & Watkins, 2000). The standardized estimates (factor loadings) of all the items representing all constructs are above 0.74, confirming the content validity requirements. Table 4 shows the values of Average Variance Extracted (AVE), Composite Reliability (CR), and the inter-correlation values between each construct with the squared root of AVE values represented in the diagonals. The AVE values are above 0.50, and this confirms the convergent validity requirements.
This helps create accurate and engaging descriptions for their large inventory. AI-generated descriptions were even better than human-written ones in tests. Stitch Fix’s approach involves both artificial intelligence and human experts, making content that keeps getting better. Furthermore, Sidekick’s capabilities mirror the ‘co-pilot’ approach promoted by artificial intelligence technology companies like OpenAI and Microsoft.
From a theoretical perspective, this research contributes to the nascent literature on AI-powered chatbots in conversational commerce both for how chatbots should be designed and for context-related deployment considerations. First, our paper contributes to emergent theories on the effects of anthropomorphism on consumer behavior and the psychological mechanisms that underlie this relationship. The current research shows that positioning chatbots powered by artificial intelligence as anthropomorphic can motivate consumers to perceive products and services recommended and purchased via chatbots as more personalized. It therefore becomes critical for marketers to reduce consumer scepticism towards chatbots and understand how to ensure positive consumer perceptions of and behavior towards the latter (Araujo, 2018; Roy & Naidoo, 2021). Mayer and Harrison (2019) emphasized the term “conversational commerce”. Conversational commerce refers to buying activity by a customer through a digital assistant.
Based on this, we expect that consumers are likely to increase the subjective value of a tailor-made product when they interact with a chatbot with anthropomorphic cues versus a chatbot without such cues. In terms of functionality, conversational AI systems aim to provide holistic, engaging interactions that make them conducive to customer service environments. They can engage in human-like dialogues, remembering past interactions and using this information to shape future responses. This ability to learn from past interactions allows them to provide personalized customer service, and to perform sales and marketing tasks more effectively. Conversational AI involves more complex systems designed to understand, process, and respond to human language in a way that is both contextual and intuitive. It goes beyond the rule-based interactions of traditional chatbots, and incorporates sophisticated machine learning algorithms to understand intent, regardless of the language or phrasing used.
Natural Language Processing (NLP) and Natural Language Understanding (NLU) are fundamental to the current wave of artificial intelligence. These fields produce complicated algorithms that let programs comprehend, interpret, and generate human language in a meaningful, contextually-appropriate way. For eCommerce companies, these tools promise to finally deliver online experiences that align seamlessly with consumer behavior. But it’s eCommerce where these conversational AIs are positioned to truly overhaul the customer experience (CX).
As consumers are increasingly turning to online purchases, eCommerce growth is predicted to continue (Tokar et al., 2021). Therefore, it is important to examine this tendency towards isolation and loneliness and the effects it will have on marketing. Specifically, we examine the potential moderating effects loneliness has on perceived product personalization and willingness to pay a higher product price in conversational commerce settings. While today’s chatbots use artificial intelligence to conduct sophisticated dialogs, earlier chatbots had a more fixed interaction style based on multiple-choice questions (Turban et al., 2018).
Product type (search or experience) could be investigated as a potential moderator. The results from study 2 confirm that anthropomorphic cues in chatbots have a positive effect on perceived product personalization (H1 was supported). However, the effect of anthropomorphic cues was not significant on the willingness to pay a higher product price (H2 was not supported). Finally, this study shows that situational loneliness moderates the effect of anthropomorphic cues on perceived product personalization (H4 was supported). The first known text-based chatbot ELIZA (Weizenbaum, 1966) was developed in the 1960s as a computer program that used natural language to simulate human-like conversation. This was followed in the 1980s by speech-based dialog systems, voice-controlled user interfaces, embodied chatbots, and social robots (McTear et al., 2016).
Microsoft also added a feature to Bing Chat where it can make images using AI through DALL-E 2. Just start a new conversation and select the more creative conversational style. Whether you’re a seasoned or beginner creative professional, writer, or web developer, AI chatbots can make your work easier.
According to CX statistics, brands that provide a high degree of personalization gain customer loyalty, which is 1.5 times higher than brands that struggle with personalization. Conversational AI leads the charge in the e-commerce space, offering a seamless solution for delivering tailored experiences across multiple devices. Master of Code’s AI chatbots for Conversational commerce supports powerful integrations with third-party tools and software enabling eCommerce companies to expand conversational connectivity. The eCommerce industry is becoming the most competitive industry all over the globe and success depends on the company’s ability to differentiate itself from the rest. And offering a unique sales experience within AI chatbots for Conversational commerce for the customer is one of the most effective ways of doing this. AI chatbot can offer a seamless and enhanced customer experience to thousands of customers daily 24/7.
Conversational AI statistics
But now, generative AI has the potential to change things further by revolutionising customer experiences. Not to mention the ways it’s also increasing productivity, driving conversions, and fostering customer loyalty. A whopping 84% of ecommerce professionals believe AI gives companies a competitive advantage.
Clients are more informed and want a fast, seamless, and smart user interface. To meet these new customer demands, brands are using AI in eCommerce to deliver personalized experiences. And Conversational AI with embedded Generative AI techniques is becoming the most effective of them all. From a customer service standpoint, time, speed, and availability are its three crucial pillars. You need to be available to your customers, at all times and provide quick resolution.
These findings align with the propositions made from uncanny valley theory that human likelihood can induce more involvement and engagement towards the object. Building upon this view, the study proposes the following hypothesis. These findings are further supported by the fact that the effect of anthropomorphic cues on perceived product personalization is more pronounced when consumers experience high (vs. low) situational loneliness.
Consumers place a premium on the ability to deliver a positive experience, as much as on the quality of your products or services. The newest AI engines, such as BoomTrain, are deployed across various consumer contact points to analyze how customers engage with online retailers. It’s done by providing data-driven insights into client preferences and behavior. Engaging with clients after purchase boosts an interest in the product and brand.
Bing Chat has evolved from a text-based approach to search and chat to a more visually comprehensive experience that includes image and video responses. Microsoft launched Bing Chat to improve search results and make it easier to answer queries using natural language. Bing Chat distinguishes itself from competitors by generating written and visual content within the Chat. Lyro provides customers with detailed responses based solely on your support content, minimizing the probability of wrong answers. Jump into ongoing conversations and provide your own answers to customer questions whenever you want. Lyro also sends you notifications whenever customers want to talk to a real person, so your team can fill in its blind spots.
The best AI tools to help you write, create videos and imagery, prompt the best hashtags and times to post, and much more. With Heyday, you can even set your chatbot up to include “Add to cart” calls to action and seamlessly direct your customers to checkout. Conversational AI solutions like Heyday make these recommendations based on what’s in the customer’s cart and their purchase inquiries (e.g., the category they’re interested in). Another fundamental component, human speech recognition technology, converts spoken language to text, allowing the system to process and comprehend the input.
Chat by Copy.ai
Scalenut is perfect for quick content creation and is the tool to use if you’re a solo writer or manage a team of writers. Writesonic is an excellent option for bloggers, marketers, and content creators who need to generate significant content. It’s particularly useful for new bloggers looking to quickly produce new content. The user interface is simple, affordable, and easy to customize, making it a great option for anyone.
Adzooma is the top choice for digital marketers, small business owners, and agencies who need AI-powered insights and dashboards to make informed decisions across marketing initiatives. Pro Rank Tracker appeals to businesses, digital marketers, and SEO professionals looking to monitor website performance, optimize content, and stay ahead of competitors in the ever-changing digital landscape. Rank Math is a favorite among website owners, bloggers, and content creators using WordPress to optimize their content for better search rankings and increased organic traffic. Alli AI offers a 10-day free trial with paid plans starting at $299 per month. Surfer SEO is ideal for digital marketers, content creators, and website owners aiming to optimize their content, boost search engine rankings, and outperform competitors in search results.
Master Tidio with in-depth guides and uncover real-world success stories in our case studies. Discover the blueprint for exceptional customer experiences and unlock new pathways for business success. When OpenAI announced ChatGPT in November 2022, it set off a frenzy in the tech industry.
We are now in the full use stage, and you may be considering using eCommerce chatbots. AI chatbots have established a strong place in every industry and eCommerce is no different. Run the numbers with our Chatbot ROI Calculator and get estimated results of the return you could get from implementing conversational Al across your business.
In this article, we will explore the background of AI chatbots, the features to look out for when choosing one, and the best conversational AI chatbot solutions for eCommerce. Retailers attempt various technology formats to maximize their conversion potential (Vukadin et al., 2019). When it comes to buying through a developed technology, competence plays a significant role. Ihtiyar and Ahmad (2014) found that intercultural communication competence and its positive impact on purchase intention. Bassellier et al. (2001) supported that technology competence can be mainly attributed to system intelligence.
It works well with apps like Slack, so you can get help while you work. Claude 3 Sonnet is able to recognize aspects of images so it can talk to you about them (as well as create images like GPT-4). Claude is a noteworthy chatbot to reference because of its unique characteristics. It offers many of the same features but has chosen to specialize in a few areas where they fall short. It has a big context window for past messages in the conversation and uploaded documents.
With integration with popular software programs such as Clickup, HubSpot, Slack, and Salesforce, Meetgeek is beneficial throughout your workflow. It provides features such as auto-join, generating automated notes and summaries, and post-meeting insights, making it a great choice for busy marketers. Airgram is an AI-powered tool that provides real-time transcription for online meetings, such as those held on Google Meet, Zoom, and Microsoft Teams.
They also appreciate its larger context window to understand the entire conversation at hand better. It helps summarize content and find specific information better than other tools like ChatGPT because it can remember more. ChatGPT should be the first thing anyone tries to see what AI can do. Two popular platforms, Shopify and Etsy, have the potential to turn those dreams into reality.
Moreover, momentarily lonely consumers interacting with an anthropomorphic chatbot would also be willing to pay a higher product price than consumers not experiencing situational loneliness. Managers would thus be well advised to consider this segment and review and enhance the effectiveness of their positioning and communication campaigns. This study aims to provide additional support for H1 and tests whether attribution of verbal anthropomorphic cues increases the willingness to pay a higher product price (H2). Prior research has argued that anthropomorphism has a positive and significant influence on product value (Hart et al., 2013) and leads to an enhanced evaluation of the product (Landwehr et al., 2011). However, it remains uncertain whether individuals would be willing to pay a higher product price for the same product if they interact with an anthropomorphic chatbot versus non-anthropomorphic.
Touching almost every aspect of marketing, it can significantly improve efficiency for individuals or teams. By seamlessly connecting your Search and Social ad platforms, Adzooma pulls in data and facilitates the launch of new campaigns. Moreover, it empowers you to maximize your ad initiatives by suggesting changes for increased ROI. Ocoya is a dream for businesses and eCommerce ventures seeking effortless social media content creation and scheduling to boost their online presence. Retention Science is designed for large eCommerce brands aiming to improve customer retention rates, foster customer loyalty, and drive growth through data-driven insights and personalized marketing efforts. They allow you to create images, logos, and vector art through a text prompt.
- In the source attractiveness model (McGuire 1985, p. 264), the effectiveness of a message depends on three components — “familiarity,” “similarity,” and “likability” of an endorser.
- However, advancements in ML and NLP have led to the development of sophisticated AI helpers.
- Then to identify what to say next in a conversation, a chatbot employs a set of predetermined rules and a decision-making tree, this process is known as dialogue management.
- E-commerce brands are also leveraging conversational AI on social media platforms to engage with customers, answer queries, and drive sales.
- Just as showcased with BloomsyBox, our expertise extends to assisting eCommerce brands in seamlessly integrating Generative AI into their chatbots, ensuring they remain pioneers in this transformative eCommerce landscape.
- In this powerful AI writer includes Chatsonic and Botsonic—two different types of AI chatbots.
Users can generate images with a text prompt, change the look and feel of vector art with recoloring, create stunning text effects, and edit existing photos. We love that Adobe’s AI is trained on royalty-free and Adobe Stock images, so there’s no worry about copyright infringement. Firefly integrates into Creative Cloud products, such as Photoshop and Illustrator, making it a useful companion for busy creatives. Pictory AI is perfect for designers, content creators, and businesses looking for an automated solution to convert long-form text and videos into engaging video content, enhancing visual storytelling. GPTZero is another great option for those looking to detect AI-generated content. Developed by a Princeton University student, it’s designed to detect AI written by LLMs at the sentence, paragraph, or document level.
Its ease of use, realistic-sounding voices, and support for 20 languages make it a great option. Play.ht users are impressed with the output, especially in languages other than English. However, some users say it may take several tries to get the AI voice where you want it, using up valuable character credits. We like Play.ht primarily for the quality (and quantity) of its AI voices. With over 900 AI voices, there’s a good chance you’ll find one you like. Plus, you can adjust pronunciations and other aspects of a generated voice to truly personalize it.
AI eCommerce chatbots and conversational apps may be instrumental in automating various order management tasks. For instance, they can ask customers to upload receipts after payment to the business’s bank account for order processing. Many chains have already implemented AI chatbots in the order process, where customers can even make their orders on Facebook messenger and other platforms. Online stores were the earliest adopters of automated interactive messaging applications from as early as 2011. The bots bore the promise of making shopping easier by solving a lot of headaches for online retailers, from providing quick answers to customer FAQs to generating leads to offering product recommendations. For product descriptions, they use fine-tuning, a process to make AI understand their style and language.
The learning curve is steeper than other tools, so coding knowledge may be required. Up first on our shortlist of the best AI website builders is Divi AI. Built on impressive AI models, Divi AI can generate and rewrite text specific to your site, create incredible images, and even generate CSS and custom code. Divi AI integrates seamlessly with Elegant Themes’ no-code Visual Builder, so you can easily build websites on the front end. Combined with Divi’s impressive Theme Builder and thousands of pre-made layouts, Divi AI provides the perfect solution for building a WordPress website fast.
We all know that ChatGPT can sound somewhat robotic when using it for writing assignments. You can foun additiona information about ai customer service and artificial intelligence and NLP. Jasper and Jasper Chat solved that issue long ago with its platform for generating text meant to be shared with customers and website visitors. These best AI tools offer a variety of solutions to improve productivity and automate workflows. To help you decide on the right tools, glance over the table to compare our top AI products by their pricing and free plan offerings. Kickresume offers a free plan with paid plans starting at $19 per month. Resume.io is regarded highly for how easy it is to create a good resume.
Chat With Sales
Previous studies have supported that emerging technology formats can support purchase intention through digital modes (Kim, 2019). Given the emergence of digital assistants, it has become increasingly possible that users have started using them for commercial purposes. It is pertinent from psychology theories that perceived animacy is built from animated cues and stimuli through artificial agents (Shultz & McCarthy, 2014). Digital assistants provide voice and speech cues with human aspects to bring human-like features. It will be interesting and valuable to investigate how these animacy features enhance purchase intention through digital assistants in a theoretical and practical context.
10 AI Chatbots to Support Ecommerce Customer Service (2023) – Shopify
10 AI Chatbots to Support Ecommerce Customer Service ( .
Posted: Tue, 28 Nov 2023 08:00:00 GMT [source]
This technology leverages natural language processing and machine learning to generate responses that are tailored to the specific needs and preferences of each customer. By providing a conversational experience that closely mimics human interaction, Generative AI in eCommerce Chatbot can enhance customer engagement and lead to higher sales conversion rates. Acting like a persevering human sales manager, a virtual assistant answers customers’ questions about products they are considering buying. Conversational AI tools such as chatbots have become ubiquitous in the customer-service industry and been found to improve service automation. A subset of artificial intelligence that empowers systems to learn and progressively improve by analyzing vast amounts of data, machine learning is a foundational element of conversational AI. Through algorithms, conversational interfaces use tools such as sentiment analysis to refine their understanding of language, adapt to user preferences, and enhance their response-generation capabilities.
Trend #2: Visual Search and Augmented Reality (AR)
This ad campaign resulted in a 25% increase in opt-ins to the brand’s subscription plan in comparison to its business-as-usual video ads. The data that support the findings of this article are available on request from the corresponding author. The data are not publicly available due to them containing information that could compromise research participant consent.
Kickresume is a great tool for job seekers who are just hitting the workforce or have limited work experience. However, those with more professional skill sets should look elsewhere. Resume.io is designed for individuals seeking standout resumes for job applications. Job seekers looking to create a professional and effective resume should give Resume.io a try. It offered a user-friendly interface, customizable designs, and a variety of pre-made templates for different industries and styles. The community says Copy.ai is great for generating and improving all types of copy but can sometimes generate inaccurate results.
Since then, researchers in computer science, information systems, human–computer interaction, and marketing have extensively explored chatbots. The recent technological advancements in NLP technology and AI allow the development of new and more efficient chatbots (Pantano & Pizzi, 2020) giving them traits that make them seem increasingly more human. Conversational AI chatbots with embedded Generative AI technology can provide a range of benefits to businesses. These chatbots can personalize product recommendations, making it easier for customers to find products that meet their needs. Additionally, AI chatbots can upsell and cross-sell products, increasing revenue for the business.
How AI Is Changing the Face of eCommerce
They prompt eCommerce stores to empathize and take action based on consumer preferences and feedback. For example, most queries often range from return policies, delivery time, shipping costs, and pricing information. Many eCommerce https://chat.openai.com/ businesses are plagued with a high volume of inquiries on their orders. While the customer has every right to know the status of their order, it’s difficult to address them when there are thousands of orders across regions.
Previous studies have supported that anthropomorphic impersonation induces purchase intention. For example, Payne et al. (2013) supported that brand anthropomorphic personalities can enhance purchase intention. To support this view, the study by Qiu and Benbasat (2009) found that the anthropomorphic recommendation agents used in e-commerce websites can aid the consumer purchase process. Guthrie (1995) found that anthropomorphic features can ease understanding of the product by familiarising the experience. Notably, Labroo et al. (2008) supported that the accumulation of humanlike experience can induce more likelihood to purchase the product. Digital assistants embed human voice-like characteristics that can induce consumers to book through digital assistants.
Pro Rank Tracker is an AI-driven search engine optimization tool that helps businesses improve their online visibility by tracking keyword rankings and providing insightful reports. Connect it with your Google Search Console (GSC) account, and it starts pulling in all the data points. Best of all, it tracks and displays ranking history so you can tell how your websites are performing over time. Rank Math is an AI-powered SEO plugin for WordPress that helps users optimize their content, insert schema markup, and drive more organic website traffic.
Better communication enhances interactions and improves the results you get from AI systems. As well as better communication improving AI responses, we can also become better communicators in general conversational ai ecommerce with the help of AI. Bing Chat and ChatGPT are both AI chatbots that use OpenAI’s GPT-4 language model. When you talk to Bing AI Chat, you ask questions differently from simple keyword searches.
The best AI platforms offer highly relevant personalisation at the individual level without having to create a separate model for each customer. By incorporating user feedback, AI-based recommendations and personalisation become smarter over time, leading to increased conversions and higher customer satisfaction. Customers aren’t the only ones who reap the benefits of AI in commerce.
As with the impact of generative AI’s large language models on the greater business world, shopper conversations with virtual assistants are providing a new dimension to the omnichannel customer experience. Through conversational commerce, businesses can increase engagement, reduce the number of abandoned carts, boost online sales and build brand loyalty. This paper stresses product personalization which can be critical in eCommerce settings; it can be highly beneficial for online merchants and their consumers.
AI eCommerce & Sales
Learn everything about digital transformation in customer service, including its definition, benefits and how it influences customer service operations. The renowned French beauty giant Sephora is at the forefront of innovation in the beauty industry, revolutionizing how customers discover and purchase products through its intelligent virtual agent, Sephora Virtual Artist. Analytics has long been essential to the growth of e-commerce brands. However, conversational AI has evolved into a predictive powerhouse, enabling e-commerce brands to forecast trends and anticipate customer needs with unprecedented accuracy. In today’s fast-paced world of online shopping, keeping up with the latest trends isn’t just a choice; it’s a must. One trend that’s causing quite a stir is conversational AI and its potential in the e-commerce space.
Bloomreach buys Radiance Commerce for AI and ‘conversational commerce’ – Digital Commerce 360
Bloomreach buys Radiance Commerce for AI and ‘conversational commerce’.
Posted: Tue, 23 Jan 2024 08:00:00 GMT [source]
In fact, most businesses implementing AI report moderate to major improvements in employee productivity and satisfaction (84% and 82%, respectively). Developing a deep working knowledge of AI tools gives employees valuable career experience with a new technology. It can also offload many job functions that once felt tedious and monotonous, opening up new career trajectories and reskilling opportunities. Happy customers are more likely to make another purchase, which can help reduce the cost of acquiring new customers that may be 5 to 25 times more expensive than retaining existing clients. For instance, the AI bot can help resolve issues revolving around the delivery status, returns, or exchanges.
The ability to find temporary comfort in an object provides people with a valuable psychological benefit (Keefer et al., 2012). When this happens, individuals may turn towards material substitutes that can be replaced quickly and provide an instant reward without much effort. Money and a preoccupation with money acts as a defense mechanism for those who feel vulnerable (Zhou & Gao, 2008) with material purchases providing a temporary sense of need satisfaction (Van Boven & Gilovich, 2003). Ashton Kutcher looks at OpenAI’s generative video tool, Sora, as the future of filmmaking. A dynamic presenter, researcher and thought leader on emerging technology best practices, Kathleen is a frequent speaker and keynoter at industry events. She helped launch the AI-focused working group at ATARC and serves as the AI working group chair, helping organizations and government agencies apply AI best practices.
Therefore, your customer should enjoy a near-perfect experience of human-like interaction. E-commerce websites are using chatbots to improve their customer support service. One of the key benefits of conversational AI for e-commerce is consumer self-service via online, mobile, and phone channels. Typically, disguised as a chatbot or speech bot, the conversational AI system reads client inquiries and attempts to react in the same manner as a human agent would.
The natural tendency for people is to seek substitutes or compensations when their basic needs are frustrated (Deci & Ryan, 2000). Prior research has shown that lonely consumers seem to compensate for high-quality social connections by buying physical products and services. When individuals experience threat of uncertainty, they aim to find alternative sources of security to protect themselves against threats to their psychological equanimity.
Play.ht offers a great free plan with paid plans starting at $39 per month. Descript offers a free plan with paid plans starting at $15 per month. Meetgeek is another excellent AI tool for transcribing your online meetings.
It allows you to focus on the meeting rather than trying to keep up with taking notes. Airgram is also an online meeting space, so you can tackle everything in one place. Winston AI offers a free plan with paid plans starting at $18 per month. It’s also very easy to use and is usually spot on, with only occasional glitches. SEO writers, content creators, or small business owners will love Wordtune. It allows you to preserve your writing style while receiving tips from AI to improve your content.
Provides new sources of data on customer behavior, language, and engagement. Create your custom online store in minutes with 10Web AI Ecommerce Website Builder and take your business online. Securing transactions and safeguarding your Chat GPT clients’ data is critical to providing a service via digital channels. A security incident management policy, data isolation, and data protection following privacy and auditing standards are essential components of excellent service.
With its advantages, best practices, and challenges, e-commerce businesses can make their brand stand out in the market with easy, data-driven, and smooth customer engagement. It not only increases the shopping experience but also creates meaningful conversations and increases user engagement. By evaluating customer data behavior and preferences, AI can provide personalized product recommendations. While the evolution of chatbots has made customer service more accessible and efficient, building a chatbot that drives results is not a walk in the park. It takes careful thought, innovative design, and regular optimization. Businesses can also integrate conversational AI with various communication channels and tools, ensuring real-time interactions across platforms.
It connects to various websites and services to gather data for the AI to use in its responses. This allows users to customize their experience by connecting to sources they are interested in. Pro users on You.com can switch between different AI models for even more control. Our next AI website chatbot, Botsonic, is brought to you by the folks at Writesonic.
Every Tidio account starts with 50 Lyro conversations, but the limit does not refresh for free. If you’re using the Lyro plan, your limit refreshes 30 days from the moment you made the payment. Lyro is not available to businesses operating in certain industries, such as medical & healthcare, gambling, investing, weapons & ammunition, and several others.
The Economic Impact of Generative AI: The future of work in Malaysia
This study marks the latest in our endeavours to evaluate the influence of this new age of AI. It indicates that generative AI is ready to revolutionise roles and improve efficiency in areas such as sales and marketing, customer service, and software creation. In doing so, it could generate trillions of dollars in value across industries from ecommerce to healthcare. For the marketing industry, our platform can help you create content, automate product description creation, craft advertising messages, and generate customer communication to improve engagement, retention, and revenue. For the entertainment industry, our generative AI technology could help your company by creating customized content with a click, producing multiple headlines, calls-to-action, real-time commentaries, summaries, and valuable statistics. The economic potential of generative AI is likely going to experience exponential growth in ways we probably haven’t considered or seen coming.
Some of this impact will overlap with cost reductions in the use case analysis described above, which we assume are the result of improved labor productivity. Generative AI is poised to impact various industries, with banking, high tech, and life sciences expected to experience significant transformations. McKinsey identifies customer operations/service, marketing and sales, software engineering, and R&D as the most valuable business functions likely to benefit from generative AI. The McKinsey report defines generative AI as applications typically built using foundation models.
I believe the time is now for businesses to think about how to capitalize on generative AI to augment workflows, gain a competitive advantage and create their ideal future. Interacting with most discriminative AI models requires the use of specific syntax or knowledge of a programming language. This takes time to adapt to and greatly limits the range of people capable of using the model. However, generative models use Natural Language Interfaces (NLIs) to interpret text as opposed to code. NLIs reduce the technical learning curve and widen the potential user base, empowering a much larger number of people to utilize the model effectively. AI has been driving value for businesses since the early 2000s; however, the majority of AI models have been discriminative, not generative.
More articles by this author
In factories where people operate complex machines and work with hazardous materials, avoiding accidents and ensuring safety are priorities. Machines and robots can perform these more laborious tasks with increased efficiency and without causing harm. As a result, companies don’t have to stress about extra costs resulting from job-related accidents, and employees can focus on other lower-risk tasks. Though generative AI will have a significant impact across all industry sectors, banking, high tech and life sciences are among the industries that could see the biggest impact on percentage of their revenues from generative AI, McKinsey said. Generative AI could add as much as $4.4 trillion annually to the global economy and will transform productivity across sectors with continued investment in the technology, according to a new study. Generative AI is one of the rare technologies powerful enough to accelerate overall economic growth — what economists call a “general-purpose technology.” These innovations have the potential to positively transform economies and societies.
- It can also enhance performance visibility across business units by integrating disparate data sources.
- AI trained on these models can perform several functions; it can classify, edit, summarize, answer questions, and draft new content, among other tasks.
- Understanding what’s coming next demands recognising the significant advancements that have paved the way for generative AI, a development that spanned decades.
This report seeks to contribute to this discussion by providing early insights and raising awareness of the economic opportunities that generative AI can create, and what it means for local industries and workforce readiness. Another approach for businesses would be to scale up by collaborating with other industry players and create a consortium or third-party platform that is not directly aligned with any single company. This would allow them to pool resources and develop a more competitive AI-driven interface that can cater to a wide range of consumer preferences.
The economic potential of generative AI: 75% of AI value comes from Customer Operations & Sales (McKinsey)
“Although the impact of AI on the labor market is likely to be significant, most jobs and industries are only partially exposed to automation and are thus more likely to be complemented rather than substituted by AI,” the authors write. In other cases, generative AI can drive value by working in partnership with workers, augmenting their work in ways that accelerate their productivity. Its ability to rapidly digest mountains of data and draw conclusions from it enables the technology to offer insights and options that can dramatically enhance knowledge work. This can significantly speed up the process of developing a product and allow employees to devote more time to higher-impact tasks. Generative AI’s evolution has been gradual, fueled by substantial investments in advanced machine learning and deep learning projects.
Having both these things requires a big increase in investment in semiconductors which in turn requires a big increase in investment in network capacity. We spoke with Briggs about how the team’s forecast has held up over the past year, which businesses are adopting generative AI, and the technology’s impact on the labor market. A report by McKinsey & Company found that AI could automate up to 45% of the tasks currently performed by retail, hospitality, and healthcare workers. While this could lead to job displacement, the report also noted that just because AI could automate a job doesn’t necessarily mean that it will, as cost, regulations, and social acceptance can also be limiting factors. Optimizing inventory management and recommending products to customers based on their purchase history and browsing behavior is only part of the value of gen AI in the retail industry.
While adoption of generative AI is lagging investment in the technology, Goldman Sachs Research sees potential for AI to automate many work tasks. It’s expected to start having a measurable impact on US GDP in 2027 and begin affecting growth in other economies around the world in the years that follow. You can foun additiona information about ai customer service and artificial intelligence and NLP. The use of gen AI in finance is expected to increase global gross domestic product (GDP) by 7%—nearly $7 trillion—and boost productivity growth by 1.5%, according to Goldman Sachs Research. Gen AI is a good fit with finance because its strength—dealing with vast amounts of data—is precisely what finance relies on to function. In the financial industry, AI algorithms detect fraud and identify investment opportunities. Generative AI has shown the potential to automate routine tasks, enhance risk mitigation, and optimize financial operations.
According to the same research by Goldman Sachs, only 7% of U.S. jobs risk automation, while 63% will leverage AI-enabled augmentation, and roughly 30% will remain unaffected. Traditional models have been trained on smaller, specialized datasets to serve a specific purpose (e.g., analyze previous machine maintenance patterns to predict when servicing is necessary). Generative AI models are trained on large databases, such as the entire publicly available internet, and so can serve a much wider range and versatility of use cases. Luxembourg can leverage its strong position on fundamental AI adoption drivers, but needs more talent and innovation to capture the potential. If you look at forecast revisions for AI hardware providers, they imply about a $250 billion increase since a year ago.
Business leaders need to bring them along by listening and addressing their concerns, and up/reskilling their employees along the way. Of CEOs surveyed by IBM, 75% believe businesses leveraging the most advanced generative AI will garner a distinct competitive advantage. The technology’s ability to widen the range of tasks AI can automate has already led to a reduction in time-consuming work and a subsequent surge in productivity.
Ernst & Young Global Limited, a UK company limited by guarantee, does not provide services to clients. AI algorithms learn from the data they are trained on, and if that data is biased or incomplete, the algorithms can perpetuate those biases in their outputs. A trial conducted at five Johns Hopkins Medicine System-affiliated healthcare facilities found that using AI algorithms to analyze medical images led to a 20% reduction in sepsis deaths in hospitals. Sepsis, which happens when the response to an infection spirals out of control, is responsible for one out of three in-hospital deaths in the United States. According to the Centers for Disease Control and Prevention, about 1.7 million adults in the U.S. develop sepsis each year, and about 350,000 of them die. Develop a deployment strategy for incorporating AI, ML, and Big Data into your organization that will take advantage of cutting-edge technologies from Penn’s Wharton Business School.
Drucker is often considered the father of modern management due to his extensive contributions to the field. Drucker’s philosophy and thoughts on management focused on people and human relationships. He taught that knowledgeable workers are the essential ingredients of the modern economy. Central to this philosophy is the view that people are an organization’s most valuable resource and that a manager’s job is preparing and freeing people to perform. This is not the same narrative we are hearing, as many people fear that artificial intelligence may take over their jobs.
Furthermore, according to the same source, this is more than the United Kingdom’s GDP of $3.07 trillion over the same period. GDP is a standardized monetary tool that measures the market’s value based on the final goods and services produced in a country over a determined time period. By now, you have probably heard of or have an idea about what this technology is and what it does. Generative AI is a subset of artificial intelligence that can be used to produce various outputs, like image, text, audio, and other forms of data. The output depends on the intended purpose of the AI model, which can be tweaked to suit the needs of individuals and organizations based on several parameters.
And because generative AI accumulates knowledge and makes it available on demand, it’s particularly effective at improving the performance of entry-level employees, helping with wage inequality. We found significant improvements in worker productivity as measured by the number of customer issues workers were able to resolve per hour. Within four months, treated agents were outperforming nontreated agents who had been on the job for over twice as long. For AI to be deployed on a widespread basis, there’s a lot of things that need to happen. First you need to have models that are powerful enough and trained appropriately so they can actually be useful in everyday work product. Then you need to have the capability to facilitate and answer all the queries that people are going to be posing to AI models, when they do use them every day multiple times a day when they’re engaged in regular work.
This implies that more than 85% of employment growth over the last 80 years is explained by the technology-driven creation of new positions, our economists write. A new wave of AI systems may also have a major impact on employment markets around the world. Shifts in workflows triggered by these advances could expose the equivalent of 300 million full-time jobs to automation, Briggs and Kodnani write. Following are four examples of how generative AI could produce operational benefits in a handful of use cases across the business functions that could deliver a majority of the potential value we identified in our analysis of 63 generative AI use cases.
This consideration creates the necessity for new regulations and legal frameworks to ensure algorithms are used ethically. Professionals must also refrain from copying content verbatim since they may receive copyright strikes. Despite the immense creativity generative AI provides, ethical considerations regarding accountability, bias, and privacy arise. For instance, can companies fully trust an AI tool to process their employees’ sensitive data safely?
The latter has propelled AI into previously unimaginable situations which has got people divided, including well respected and highly regarded professionals in technology. It makes me (Tom Allen) laugh when people think they have got the answer for what its use will mean. When you might have got a solution for how to use Generative AI figured out, not what the eventual outcome will be as it changing every second of every day. While generative AI is an exciting and rapidly advancing technology, the other applications of AI discussed in our previous report continue to account for the majority of the overall potential value of AI. Traditional advanced-analytics and machine learning algorithms are highly effective at performing numerical and optimization tasks such as predictive modeling, and they continue to find new applications in a wide range of industries. However, as generative AI continues to develop and mature, it has the potential to open wholly new frontiers in creativity and innovation.
Applications of Generative AI
While the economic potential of generative AI is valid, its implementation may prove challenging for many companies. Professionals with remarkable technical expertise must be recruited so they can operate the algorithm effectively. Therefore, many organizations that can’t afford such additions may be left behind and make massive efforts to catch up to their competition. While traditional manual labor positions may fall into obscurity or decrease significantly, other, more technical jobs will be created. However helpful and life-saving AI-powered machines may be, they can’t operate on their own.
The algorithm can monitor everyone’s performance, provide feedback, notice skill gaps, and advise on development opportunities. Many organizations across the globe are now using AI tools to create content for recruitment as HR benefits highly during acquisition and onboarding. They ask the algorithm to create job postings based on skills, keywords, and older listings. In advanced cases, companies may design avatars for each candidate and provide personalized feedback. The rapid development of generative AI also has the potential to “change the anatomy of work” and can automate work activities that absorb 60 to 70 per cent of employees’ time today.
Now, the generative AI market is expected to grow from $40 billion in 2022 to $1.3 trillion over the next 10 years. In this article, I aim to demystify how generative AI constitutes a distinct revolution and explore the prospective economic impacts of deploying this technology across diverse sectors. In fact, if we look at the labor demand that is generated it’s probably driven a net increase in employment. And so, it’s very well possible, and probably even likely, that the net impact on the labor market has been positive thus far.
Managing through the generative AI revolution will involve diving into the most relevant use cases, evaluating a strategic approach to leveraging AI tools, and re-skilling the workforce to match changing demand. Overall, generative AI presents both challenges and opportunities, and organizations must be prepared to navigate the changing landscape to stay ahead of the game. Generative AI is only a piece of the pie organizations should consider in context of the value AI can generate.
These matters should be addressed early on and companies must devise plans to effectively treat the gray areas. Excitement over this technology is palpable, and early pilots are compelling,” the McKinsey report said. Ahead of the meeting, major AI companies, including Microsoft and Alphabet’s Google, committed to participating in the independent public evaluation of their systems.
Specifically, AI-powered personal assistants like Siri, Alexa, and Google Assistant can now understand and respond to complex requests, making it possible to deliver on the promise of truly personalized assistance. As AI continues to advance, these personal assistants will become even more sophisticated, learning individual preferences and providing tailored recommendations, while also generalizing to a broader set of tasks. This shift in interaction modality has significant implications for businesses, as it means consumers may no longer be directly influenced by traditional advertising methods. Instead, advertisers may need to target the AI agents themselves, which will be responsible for surfacing brands to their users. Generative AI represents the next frontier of productivity and revenue growth in the IT and software development industries.
From specialized to generalized intelligence: The pathway paved by generative AI models
Generative AI could enable labor productivity growth of 0.1 to 0.6 percent annually through 2040, depending on the rate of technology adoption and redeployment of worker time into other activities. Combining generative AI with all other technologies, work automation could add 0.2 to 3.3 percentage points annually to productivity growth. However, workers will need support in learning new skills, and some will change occupations. If worker transitions and other risks can be managed, generative AI could contribute substantively to economic growth and support a more sustainable, inclusive world.
Around the same time, Variational Autoencoders (VAEs) and Recurrent Neural Networks (RNNs) began to demonstrate their ability to generate novel content. In 2012, the McKinsey Global Institute (MGI) estimated that knowledge workers spent about a fifth of their time, or one day each work week, searching for and gathering information. If generative AI could take on such tasks, increasing the efficiency and effectiveness of the workers doing them, the benefits would be huge.
Goldman Sachs estimates that generative AI could automate tasks that take up to one-fourth of employees’ time today. These assessments have sparked concerns about job displacement and an uncertain future of work. No, it really hasn’t changed because our forecasts don’t assume any AI boost at all before 2027. Even though we do still think that it’s going to be a significant driver of productivity and GDP growth over a much longer horizon.
Discriminative models excel at making predictions from existing data and identifying anomalies. These models power everything from social media content recommendation engines to financial fraud detection platforms. I think that a lot these reasons broadly reflect that companies want to make sure that they get generative AI right, and companies are therefore taking deliberate approach to AI adoption.
AI-powered security solutions can analyze large datasets, detect patterns and anomalies, and suggest countermeasures before cybercriminals strike. AI has permeated our lives incrementally, through everything from the tech powering our smartphones to autonomous-driving features on cars to the tools retailers use to surprise and delight consumers. Clear milestones, such as when AlphaGo, an AI-based program developed by DeepMind, defeated a world champion Go player in 2016, were celebrated but then quickly faded from the public’s consciousness. We would just add that generative AI is just that, in the business of generating something, usually to see, although there are aspects such as generative code and other similar offerings. The quiet technology revolution is happening in predictive and analytics AI, where hyper-personalisation for each consumer’s product selections applicable to the immediate needs and maximum relevancy is quietly taking over. And situations like this are likely going to become a reality for companies in various sectors of different sizes.
First and foremost, we see adoption rates higher in areas like information services, finance and insurance. The motion picture and sound recording industry, for instance, is another area where adoption is far above the economy wide average. “That being said, the early signals of future productivity gains look very, very positive,” he adds.
To harness generative AI’s potential, significant investments in workforce adaptation are essential. This includes reskilling workers and managing transitions as certain roles evolve or disappear. If managed well, generative AI could boost labor productivity by 0.1 to 0.6 percent annually through 2040 and combine with other technologies to enhance overall productivity growth by 0.5 to 3.4 percentage points annually. Malaysia’s digital economy has experienced rapid growth, with the ICT sector contributing 22.6% to Malaysia’s GDP in 2021, driven by government initiatives, private sector investment, and increasing adoption of digital technologies. The National Fourth Industrial Revolution (4IR) Policy document identifies AI as one of the key technologies that ‘’are foundational to the nation’s 4IR agenda’’ and stresses the need to develop ethical use of AI for transforming the economy.
As computational power increased, deep learning algorithms became increasingly successful, leading to an explosion of interest in AI in the 2010s. The adoption of generative AI is expected to significantly impact various industries and job markets, including manufacturing, healthcare, retail, transportation, and finance. While it is likely to lead https://chat.openai.com/ to increased efficiency and productivity, it is also expected to lead to job displacement for some workers. Generating new content based on cumulative data input makes gen AI worthwhile in many industries. The speed with which this technology can create content can help employees develop more content in less time and/or work more efficiently.
While generative AI will impact a wide variety of industries, 75% of its potential value spans just four sectors. When you combine the broader capabilities of generative models with the democratization of access provided by NLIs, the explosive rise of ChatGPT and massive generative AI market predictions become more understandable. The adoption and usage will occur when these pieces are in place, and companies actually start using AI on an everyday basis. We see about 5% of companies reporting that they do use generative AI today in regular production, but this is a fairly small share relative to the overall number of companies that we think will ultimately benefit. And ultimately, that’s going to require an increase in electricity and collective power investment to support the increase in demand that facilitating queries will require. There are several actions that could trigger this block including submitting a certain word or phrase, a SQL command or malformed data.
Companies such as Tesla and Toyota are leveraging AI-driven simulations and generative design algorithms to create lighter, more fuel-efficient vehicles with enhanced safety features. As the automotive industry transitions towards electric and autonomous vehicles, generative AI will play a pivotal role in shaping the future of transportation. While much is unknown about how generative AI will influence the world economy and society, and it will take time to play out, there are clear signs that the effects could be profound.
Such feelings, in turn, could lead to decreasing engagement and productivity, and higher turnover. Learn more about the overall report on The economic opportunity of generative AI in D9+ and get links to all country reports. These views generally align with what we’ve seen in some of the business surveys, where CEOs are asked about their intention to use generative AI. Very few say that they expect it’s going to significantly impact their business over the next one to three years. EY refers to the global organization, and may refer to one or more, of the member firms of Ernst & Young Global Limited, each of which is a separate legal entity.
If we’re talking about the things that people say that they’re using it for, marketing, automation, chatbot, speech text, and data analysis are all areas that stand out as ways that companies are applying AI right now. This is kind of the low hanging fruit where AI is most applicable, at least in its current form. Ultimately, we think that a broader set of tasks are going to be automated by generative AI.
With the help of these advanced models, creative tasks can now be automated, diversified, and customized, leading to an overall enhancement of quality. This could have massive implications for all forms of digital content creation, from social media and user generated content to digital movie or game production. If the benefits of scaling up AI models reach the point of diminishing returns, then the restrictions on China’s AI development may become less effective.
The ServiceBot streamlines processes from order tracking to client information gathering. It handles service queries efficiently, integrates with the ERP and powers customer portals, ensuring a seamless service experience. We have seen that AI-powered conversational commerce can reduce customer service costs by about 30%. According to a research, AI-powered consulting and training tools can suggest optimal consulting solutions that increase ROI by 175%. AI-powered tools can suggest optimal consulting solutions, provide feedback, and suggest areas of improvement, and even provide personalized training.
For instance, generative AI could add $200 billion to $340 billion annually to the banking sector alone. The sudden emergence of AI chatbot ChatGPT and other tools have jump-started investment in the AI sector. More than $2 billion worth of investments were made in generative AI sector in 110 deals in 2022 alone, according to Goldman Sachs. The latest estimate is an upgrade from 2017 when the consultancy estimated AI to deliver $9.5 trillion to $15.4 trillion in economic value. Artificial intelligence (AI) is rapidly enabling solutions to the challenges we face in our lives. The term was coined in 1956, but the field has only recently begun having significant effects on the economy.
Foundation models, a key component of generative AI, process large and varied sets of unstructured data, enabling them to perform diverse tasks such as classification, editing, summarization, and content generation. Issues of data privacy, security, and ethical considerations around AI-generated content need to be addressed. Ensuring the economic potential of generative ai that AI systems are transparent and that their outputs are fair and unbiased is crucial for gaining public trust and maximizing the benefits of these technologies. Generative AI, characterized by its ability to create new content—whether text, images, music, or even complex data models—heralds a new era of creativity and innovation.
The financial services and investment banking sector is maximising how end users can make their money and assets do more for them. Global banking institutions such as Goldman Sachs and JPMorgan Chase are using AI-powered algorithms to optimize trading strategies and risk management processes. By analyzing vast amounts of financial data in real-time, these companies can make more informed investment decisions and mitigate potential risks, leading to increased profitability and market competitiveness. The implications of generative AI extend far beyond the confines of academia and research labs with the technology having real actions on modern society and how we interact, do business, chat to friends, spend our time, and everything else.
In April, Goldman Sachs said the sector could drive a 7 per cent – or almost $7 trillion – increase in global GDP and lift productivity growth by 1.5 percentage points over a 10-year period. As AI continues to advance, businesses must be prepared to navigate the challenges and opportunities that come with this transformative technology. Census Bureau, there has only been one job throughout all time that has been fully automated—the elevator operator. While initially seen as job-killers for bank tellers, the adoption of ATMs led to more bank branches opening and even more roles for bank tellers in a phenomenon known as Jevon’s paradox. This is because the cost for opening a single branch dropped substantially due to automation. While large language model (LLM) technology has large potential for value generation, without strategic planning and investment, machine learning (ML) AI projects will likely fail.
“Generative AI can streamline business workflows, automate routine tasks and give rise to a new generation of business applications,” Kash Rangan, senior U.S. software analyst in Goldman Sachs Research, writes in the team’s report. The technology is making inroads in business applications, improving the day-to-day efficiency of knowledge workers, helping scientists develop drugs faster and accelerating the development of software code, among other things. Our second lens complements the first by analyzing generative AI’s potential impact on the work activities required in some 850 occupations. We modeled scenarios to estimate when generative AI could perform each of more than 2,100 “detailed work activities”—such as “communicating with others about operational plans or activities”—that make up those occupations across the world economy. This enables us to estimate how the current capabilities of generative AI could affect labor productivity across all work currently done by the global workforce. These are the result of huge investments in advanced machine learning and deep learning projects.
- EY is a global leader in assurance, consulting, strategy and transactions, and tax services.
- One-third of all entry-level roles could be automated; at the same time, junior employees armed with generative AI may potentially replace their first-line managers, leaving a vacuum in the middle of the job pyramid.
- He taught that knowledgeable workers are the essential ingredients of the modern economy.
- This leads to more efficient utilization of resources, cost savings, and increased overall IT operational performance.
- In the process, it could unlock trillions of dollars in value across sectors from banking to life sciences.
Many of the most important debates about access and control of AI systems are downstream of the scale-up vs. scale-down debate, including the debate about open-source vs. closed-source AI. Generative AI’s impact on productivity could add trillions of dollars in value to the global economy and according to McKinsey and it is already having a significant impact across all industry sectors. Generative AI is a branch of artificial intelligence that focuses on creating new and original content, such as images, text, or even code, using models trained on vast amounts of existing data. It goes beyond traditional AI techniques by enabling machines to generate creative and innovative outputs.
Goldman Sachs Research predicted last year that generative AI could boost GDP and raise labor productivity growth over the coming decade. Since publishing that outlook, investment in generative AI has boomed, but it will take time for the technology to filter into the overall economy. Generative AI has the potential to automate certain tasks, displacing some workers, and it can also create new jobs and industries.
But that probably requires a build out of an application layer to support the broader automation we see possible. We provide customized education solutions to upskill individuals and businesses to thrive in the creator economy. Our courses range from various creative segments taught by worldwide content creators, to latest creative technologies and tools including topics like GenAI tools. A study by the World Economic Forum found that adopting AI could lead to a net increase in jobs in some industries, particularly those that require higher levels of education and skills. However, the report also warned that the benefits of AI could be unevenly distributed, with some workers and regions experiencing more significant job displacement than others. In the entertainment industry, gen AI creates personalized recommendations for movies, TV shows, and music based on individual preferences.
The technology enables businesses to automate content creation, from writing compelling articles to designing engaging visuals. With personalized content becoming increasingly important, generative AI algorithms can analyze user preferences and deliver tailor-made experiences. This level of customization not only enhances user satisfaction but also drives customer loyalty and revenue growth. As we stand on the cusp of a new year, the buzz surrounding generative artificial intelligence (AI) is reaching a crescendo. The year 2024 promises to be a groundbreaking period for businesses and economies worldwide, as the economic potential of generative AI takes center stage.
Understanding what’s coming next demands recognising the significant advancements that have paved the way for generative AI, a development that spanned decades. For the context of this report, we’re referring to generative AI as the technology often developed with the help of foundational models. These foundational models are made up of complex artificial neural networks, modeled after the trillions of neurons found in the human brain. In pharma and medical products, the total potential gains from the use of generative AI could be as high as $110 billion. The main areas where the revenue increases occur in the life sciences industry include research and drug discovery, content and document generation, and contract creation.
AI is showing ‘very positive’ signs of eventually boosting GDP and productivity – goldmansachs.com
AI is showing ‘very positive’ signs of eventually boosting GDP and productivity.
Posted: Mon, 13 May 2024 07:00:00 GMT [source]
By working together, businesses can create a more level playing field and ensure they are not left at the mercy of a single dominant player in the market. While generative AI will likely not lead to a decrease in jobs, it will change the employment landscape. There will be less need for certain human input as tasks that once took hours and many hands can be completed nearly instantaneously. Managing through the generative AI revolution will involve re-skilling the workforce to match changing demand. Generative AI’s capacity to automate tasks core to a wide range of industries has led to a general fear that it will have a depressive effect on jobs. The Technology & Society Visiting Fellowship brings experts to Google to proactively engage in critical societal discussions on the ramifications of cutting-edge technology and its impact on the world.
Our analysis of 16 business functions identified just four—customer operations, marketing and sales, software engineering, and research and development—that could account for approximately 75 percent of the total annual value from generative AI use cases. “Examples include generative AI’s ability to support interactions with customers, generate creative content for marketing and sales and draft computer code based on natural-language prompts, among many other tasks,” the report said. Generative AI has opened the door to more possibilities and is expected Chat GPT to play a role in tasks requiring creativity, curiosity, and looking at information differently. Therefore, the potential of generative AI lies in its ability to enable people to achieve greater creativity, effectiveness, and efficiency in their work. This technology is already delivering large productivity gains, which will increase and spread as people and organizations come up with complementary innovations that leverage generative AI’s capabilities. As a result, overall productivity will improve, resulting in an acceleration of economic growth.
In the process, it could unlock trillions of dollars in value across sectors from banking to life sciences. Several studies and analyses have examined the impact of generative AI on the economy, with estimates ranging from $14 trillion to $15.7 trillion in economic contribution by 2030. The potential economic benefits of generative AI include increased productivity, cost savings, new job creation, improved decision making, personalization, and enhanced safety.
Today’s computing ecosystem – and, by extension, its energy consumption – is centralized in large cloud data centers. From 2010 to 2018 global computing output in data centers jumped six-fold while energy consumption rose only 6%. This relative efficiency reflects a concerted effort by cloud computing players and data center operators to optimize energy usage and performance.
How to Setup Streamlabs Chatbot Commands The Definitive Guide
Uptime commands are common as a way to show how long the stream has been live. It is useful for viewers that come into a stream mid-way. Uptime commands are also recommended for 24-hour streams and subathons to show the progress. If you wanted the bot to respond with a link to your discord server, for example, you could set the command to ! Discord and add a keyword for discord and whenever this is mentioned the bot would immediately reply and give out the relevant information. If a command is set to Chat the bot will simply reply directly in chat where everyone can see the response.
Don’t forget to check out our entire list of cloudbot variables. Use these to create your very own custom commands. Streamlabs Chatbot Commands are the bread and butter Chat GPT of any interactive stream. With a chatbot tool you can manage and activate anything from regular commands, to timers, roles, currency systems, mini-games and more.
If you want to learn more about what variables are available then feel free to go through our variables list HERE. Once you have done that, it’s time to create your first command. Streamlabs has made going live from a mobile device easier than ever before. Check out Ultra for Streamlabs Mobile to learn how to stream straight from your phone with style. If you’re brand new to Streamlabs, great news, setting up a Streamlabs ID is super simple! You can create a Streamlabs ID from Streamlabs, Cross Clip, Talk Studio, Video Editor, and Link Space.
Streamlabs Chatbot Commands: Counters
Unlike commands, keywords aren’t locked down to this. You don’t have to use an exclamation point and you don’t have to start your message with them and you can even include spaces. Keywords are another alternative way to execute the command except these are a bit special. Commands usually require you to use an exclamation point and they have to be at the start of the message. The Global Cooldown means everyone in the chat has to wait a certain amount of time before they can use that command again. If the value is set to higher than 0 seconds it will prevent the command from being used again until the cooldown period has passed.
Streamlabs chatbot allows you to create custom commands to help improve chat engagement and provide information to viewers. Commands have become a staple in the streaming community and are expected in streams. If you are unfamiliar, adding a Media Share widget gives your viewers the chance to send you videos that you can watch together live on stream. This is a default command, so you don’t need to add anything custom. Go to the default Cloudbot commands list and ensure you have enabled ! The cost settings work in tandem with our Loyalty System, a system that allows your viewers to gain points by watching your stream.
User variables function as global variables, but store values per user. Global variables allow you to share data between multiple actions, or even persist it across multiple restarts of Streamer.bot. Arguments only persist until the called action finishes execution and can not be referenced by any other action.
If these parameters are in the
command it expects them to be there if they are not entered the command will not post. In the above example, you can see hi, hello, hello there and hey as keywords. If a viewer were to use any of these in their message our bot would immediately reply. Hugs — This command is just a wholesome way to give you or your viewers a chance to show some love in your community. So USERNAME”, a shoutout to them will appear in your chat.
Cloudbot 101 — Custom Commands and Variables (Part Two)
If possible, try to stick to only ONE chatbot tool. Otherwise, you will end up duplicating your commands or messing up your channel currency. You can foun additiona information about ai customer service and artificial intelligence and NLP. Promoting your other social media accounts is a great way to build your streaming community. Your stream viewers are likely to also be interested in the content that you post on other sites.
If you have a Streamlabs tip page, we’ll automatically replace that variable with a link to your tip page. Now click “Add Command,” and an option to add your commands will appear. streamlabs variables This is useful for when you want to keep chat a bit cleaner and not have it filled with bot responses. The Reply In setting allows you to change the way the bot responds.
Stuck between Streamlabs Chatbot and Cloudbot? Find out how to choose which chatbot is right for your stream. Cheat sheet of chat command for stream elements, stream labs and nightbot.
Make sure to use $userid when using $addpoints, $removepoints, $givepoints parameters. As a streamer you tend to talk in your local time and date, however, your viewers can be from all around the world. When talking about an upcoming event it is useful to have a date command so users can see your local date. A hug command will allow a viewer to give a virtual hug to either a random viewer or a user of their choice. Streamlabs chatbot will tag both users in the response.
You can have the response either show just the username of that social or contain a direct link to your profile. Having a lurk command is a great way to thank viewers who open the stream even if they aren’t chatting. A lurk command can also let people know that they will be unresponsive in the chat for the time being.
Typically social accounts, Discord links, and new videos are promoted using the timer feature. Before creating timers you can link timers to commands via the settings. This means that whenever you create a new timer, a command will also be made for it. Shoutout commands allow moderators to link another streamer’s channel in the chat. Typically shoutout commands are used as a way to thank somebody for raiding the stream. We have included an optional line at the end to let viewers know what game the streamer was playing last.
This will display the song information, direct link, and the requester names for both the current as well as a queued song on YouTube. This will display all the channels that are currently hosting your channel. This command will help to list the top 5 users who spent the maximum hours in the stream. Using this command will return the local time of the streamer. Sound effects can be set-up very easily using the Sound Files menu.
You will need to have Streamlabs read a text file with the command. The text file location will be different for you, however, we have provided an example. Each 8ball response will need to be on a new line in the text file. An Alias allows your response to trigger if someone uses a different command.
In the picture below, for example, if someone uses ! Customize this by navigating to the advanced section when adding a custom command. Whether you’re a brand new Streamlabs creator or have been with us for years, Streamlabs ID makes it easier than ever to create content to share with the world. With Streamlabs ID you get access to Streamlabs Desktop, Mobile, Web Suite, and Console plus Cross Clip, Talk Studio and Video Editor.
Cloudbot 101 — Custom Commands and Variables (Part One)
This will give an easy way to shoutout to a specific target by providing a link to their channel. This will display the last three users that followed your channel. This will return how much time ago users followed your channel.
Below are the most commonly used commands that are being used by other streamers in their channels. If you want to take your Stream to the next level you can start using advanced commands using your own scripts. Twitch now offers an integrated poll feature that makes it soooo much easier for viewers to get involved.
Do this by adding a custom command and using the template called ! To add custom commands, visit the Commands section in the Cloudbot dashboard. Streamlabs Chatbot can join your discord server to let your viewers know when you are going live by automatically announce when your stream goes live….
Like the current song command, you can also include who the song was requested by in the response. Variables are sourced from a text document stored on your PC and can be edited at any time. Each variable will need to be listed on a separate line. Feel free to use our list as a starting point for your own. Similar to a hug command, the slap command one viewer to slap another. The slap command can be set up with a random variable that will input an item to be used for the slapping.
Streamlabs Chatbot Commands: Currency
This will return the latest tweet in your chat as well as request your users to retweet the same. Make sure your Twitch name and twitter name should be the same to perform so. This will return the date and time for every particular Twitch account created. A betting system can be a fun way to pass the time and engage a small chat, but I believe it adds unnecessary spam to a larger chat.
Once it expires, entries will automatically close and you must choose a winner from the list of participants, available on the left side of the screen. Chat commands and info will be automatically be shared in your stream. Displays the target’s id, in case of Twitch it’s the target’s name in lower case characters. Make sure to use $targetid when using $addpoints, $removepoints, $givepoints parameters. An 8Ball command adds some fun and interaction to the stream. With the command enabled viewers can ask a question and receive a response from the 8Ball.
Streamlabs will source the random user out of your viewer list. When streaming it is likely that you get viewers from all around the world. A time command can be helpful to let your viewers know what your local time is. As a streamer, you always want to be building a community. Having a public Discord server for your brand is recommended as a meeting place for all your viewers. Having a Discord command will allow viewers to receive an invite link sent to them in chat.
To return the date and time when your users followed your channel. To list the top 5 users having most points or currency. This command will return the time-duration of the stream and will return offline if the stream is not live. Make use of this parameter when you just want
to output a good looking version of their name to chat.
Make sure to use $touserid when using $addpoints, $removepoints, $givepoints parameters. Timers are commands that are periodically set off without being activated. You can use timers to promote the most useful commands.
Keep reading for instructions on getting started no matter which tools you currently use. All you need to simply log in to any of the above streaming platforms. It automatically optimizes all of your personalized settings to go live. This streaming tool is gaining popularity because of its rollicking experience.
We’ll walk you through how to use them, and show you the benefits. Today we are kicking it off with a tutorial for Commands and Variables.
The added viewer is particularly important for smaller streamers and sharing your appreciation is always recommended. If you are a larger streamer you may want to skip the lurk command to prevent spam in your chat. Displays the user’s id, in case of Twitch it’s the user’s name in lower case characters.
A current song command allows viewers to know what song is playing. This command only works when using the Streamlabs Chatbot song requests feature. If you are allowing stream viewers to make song suggestions then you can also add the username of the requester to the response. In part two we will be discussing https://chat.openai.com/ some of the advanced settings for the custom commands available in Streamlabs Cloudbot. If you want to learn the basics about using commands be sure to check out part one here. Shoutout — You or your moderators can use the shoutout command to offer a shoutout to other streamers you care about.
- Arguments only persist until the called action finishes execution and can not be referenced by any other action.
- Streamlabs Chatbot Commands are the bread and butter of any interactive stream.
- This guide will teach you how to adjust your IPv6 settings which may be the cause of connections issues.Windows1) Open the control panel on your…
Watch time commands allow your viewers to see how long they have been watching the stream. It is a fun way for viewers to interact with the stream and show their support, even if they’re lurking. And 4) Cross Clip, the easiest way to convert Twitch clips to videos for TikTok, Instagram Reels, and YouTube Shorts.
Learn more about the various functions of Cloudbot by visiting our YouTube, where we have an entire Cloudbot tutorial playlist dedicated to helping you. Next, head to your Twitch channel and mod Streamlabs by typing /mod Streamlabs in the chat. Set up rewards for your viewers to claim with their loyalty points. Check out part two about Custom Command Advanced Settings here. In this new series, we’ll take you through some of the most useful features available for Streamlabs Cloudbot.
If you aren’t very familiar with bots yet or what commands are commonly used, we’ve got you covered. To get started, all you need to do is go HERE and make sure the Cloudbot is enabled first. It’s as simple as just clicking on the switch.
A user can be tagged in a command response by including $username or $targetname. The $username option will tag the user that activated the command, whereas $targetname will tag a user that was mentioned when activating the command. Following as an alias so that whenever someone uses ! Following it would execute the command as well. If one person were to use the command it would go on cooldown for them but other users would be unaffected.
All you have to do is to toggle them on and start adding SFX with the + sign. From the individual SFX menu, toggle on the “Automatically Generate Command.” If you do this, typing ! Cheers, for example, will activate the sound effect. As the name suggests, this is where you can organize your Stream giveaways. Streamlabs Chatbot allows viewers to register for a giveaway free, or by using currency points to pay the cost of a ticket. Viewers can use the next song command to find out what requested song will play next.
Using this amazing tool requires no initiation charges, but, when you go with a prime plan, you will be charged in a monthly cycle. I would recommend adding UNIQUE rewards, as well as a cost for redeeming SFX, mini games, or giveaway tickets, to keep people engaged. If you choose to activate Streamlabs points on your channel, you can moderate them from the CURRENCY menu. You can tag a random user with Streamlabs Chatbot by including $randusername in the response.
To share variables across multiple actions, or to persist them across restarts, you can store them as Global Variables. Similar to the above one, these commands also make use of Ankhbot’s $readapi function, however, these commands are exhibited for other services, not for Twitch. This command runs to give a specific amount of points to all the users belonging to a current chat.
Again, depending on your chat size, you may consider adding a few mini games. Some of the mini-games are a super fun way for viewers to get more points ! You can add a cooldown of an hour or more to prevent viewers from abusing the command. Some commands are easy to set-up, while others are more advanced. We will walk you through all the steps of setting up your chatbot commands.
You can use subsequent sub-actions to populate additional arguments, or even manipulate existing arguments on the stack. Demonstrated commands take recourse of $readapi function. To begin so, and to execute such commands, you may require a multitude of external APIs as it may not work out to execute these commands merely with the bot. Streamlabs Chatbot is developed to enable streamers to enhance the users’ experience with rich imbibed functionality.
We’ll walk you through the process from Streamlabs, but the steps are similar from any of the sites. Get started with a Streamlabs ID to access the full suite of Streamlabs creator tools with one simple login. These variables can be utilized in most sub-action configuration text fields. The argument stack contains all local variables accessible by an action and its sub-actions. This command will demonstrate all BTTV emotes for your channel.
Latent Semantic Analysis: An Approach to Understand Semantic of Text IEEE Conference Publication
It was often promoted by BI vendors as a way to help companies build purpose-built dashboards, and it was both rigid and complex. A knowledge graph-powered semantic layer is capable of providing numerous points of view at the same time and can model complex relationships even if the data is big, siloed, and/or changing. Semantic analysis is a crucial component of natural language processing (NLP) that concentrates on understanding the meaning, interpretation, and relationships between words, phrases, and sentences in a given context. It goes beyond merely analyzing a sentence’s syntax (structure and grammar) and delves into the intended meaning.
I will explore a variety of commonly used techniques in semantic analysis and demonstrate their implementation in Python. By covering these techniques, you will gain a comprehensive understanding of how semantic analysis is conducted and learn how to apply these methods effectively using the Python programming language. This integration of world knowledge can be achieved through the use of knowledge graphs, which provide structured information about the world. One approach to address this challenge is through the use of word embeddings that capture the different meanings of a word based on its context. Another approach is through the use of attention mechanisms in the neural network, which allow the model to focus on the relevant parts of the input when generating a response.
ESWC 15 Challenge on Concept-Level Sentiment Analysis
For example, analyze the sentence “Ram is great.” In this sentence, the speaker is talking either about Lord Ram or about a person whose name is Ram. That is why the job, to get the proper meaning of the sentence, of semantic analyzer is important. Through semantic enrichment, SciBite enables unstructured documents to be converted to RDF, providing the high quality, contextualised data needed for subsequent semantic analytics discovery and analytics to be effective. Semantic analysis allows advertisers to display ads that are contextually relevant to the content being consumed by users. This approach not only increases the chances of ad clicks but also enhances user experience by ensuring that ads align with the users’ interests. In many companies, these automated assistants are the first source of contact with customers.
Stavrianou et al. [15] present a survey of semantic issues of text mining, which are originated from natural language particularities. This is a good survey focused on a linguistic point of view, rather than focusing only on statistics. Today we will be exploring how some of the latest developments in NLP (Natural Language Processing) can make it easier for us to process and analyze text. We can any of the below two semantic analysis techniques depending on the type of information you would like to obtain from the given data. As we discussed, the most important task of semantic analysis is to find the proper meaning of the sentence. This article is part of an ongoing blog series on Natural Language Processing (NLP).
Zeta Global is the AI-powered marketing cloud that leverages proprietary AI and trillions of consumer signals to make it easier to acquire, grow, and retain customers more efficiently. Create individualized experiences and drive outcomes throughout the customer lifecycle. Semantic analysis makes it possible to bring out the uses, values and motivations of the target. Semantic analysis, on the other hand, is crucial to achieving a high level of accuracy when analyzing text.
Semantic Analysis of Natural Language captures the meaning of the given text while taking into account context, logical structuring of sentences and grammar roles. MonkeyLearn makes it simple for you to get started with automated semantic analysis tools. Using a low-code UI, you can create models to automatically analyze your text for semantics and perform techniques like sentiment and topic analysis, or keyword extraction, in just a few simple steps.
Text Analytics + Semantic Enrichment products
Learn how to use Explicit Semantic Analysis (ESA) as an unsupervised algorithm for feature extraction function and as a supervised algorithm for classification. By moving from columns to concept, not only are insights accelerated, but decision-makers can also use data from any point in the value chain and then experience their benefits. With numerous teams of researchers working independently to develop new treatments, data was often siloed within teams, making it difficult to link targets, genes, and disease data across different parts of the company.
While these models are good at understanding the syntax and semantics of language, they often struggle with tasks that require an understanding of the world beyond the text. This is because LLMs are trained on text data and do not have access to real-world experiences or knowledge that humans use to understand language. LLMs use a type of neural network architecture known as Transformer, which enables them to understand the context and relationships between words in a sentence.
This cognitive instrument allows an individual to distinguish apples from the background and use them at his or her discretion; this makes corresponding sensual information useful, i.e. meaningful for a subject81,82,83,84. Registry of such meaningful, or semantic, distinctions, usually expressed in natural language, constitutes a basis for cognition of living systems85,86. Alternatives of each semantic distinction correspond to the alternative (eigen)states of the corresponding basis observables in quantum modeling introduced above. In “Experimental testing” section the model is approbated in its ability to simulate human judgment of semantic connection between words of natural language. Positive results obtained on a limited corpus of documents indicate potential of the developed theory for semantic analysis of natural language. Thus, as and when a new change is introduced on the Uber app, the semantic analysis algorithms start listening to social network feeds to understand whether users are happy about the update or if it needs further refinement.
It is possible because the terms “pain” and “killer” are likely to be classified as “negative”. Semantic analysis can be beneficial here because it is based on the whole context of the statement, not just the words used. As you can see, this approach does not take into account the meaning or order of the words appearing in the text. Moreover, in the step of creating classification models, you have to specify the vocabulary that will occur in the text. — Additionally, the representation of short texts in this format may be useless to classification algorithms since most of the values of the representing vector will be 0 — adds Igor Kołakowski.
These visualizations help identify trends or patterns within the unstructured text data, supporting the interpretation of semantic aspects to some extent. It is a crucial component of Natural Language Processing (NLP) and the inspiration for applications like chatbots, search engines, and text analysis tools using machine learning. Powerful semantic-enhanced machine learning tools will deliver valuable insights that drive better decision-making and improve customer experience. Automatically classifying tickets using semantic analysis tools alleviates agents from repetitive tasks and allows them to focus on tasks that provide more value while improving the whole customer experience.
No matter what industry you’re in, Semantic AI’s technology can redefine the way you visualize, interact with, analyze, and understand data. All rights are reserved, including those for text and data mining, AI training, and similar technologies. Meaning representation can be used to reason for verifying what is true in the world as well as to infer the knowledge from the semantic representation. The main difference between them is that in polysemy, the meanings of the words are related but in homonymy, the meanings of the words are not related.
How Does a Semantic Layer Platform Work?
Its primary purpose is to simplify data access and analysis by providing a business-friendly view of the data, hiding the complexities of the underlying data structure and technical details. But semantic analysis is already being used to figure out how humans and machines feel and give context and depth to their words. The grammatical analysis and recognition connection between words in a given context enables algorithms to comprehend and interpret phrases, sentences, and all forms of data.
The goal is to develop a general-purpose tool for analysing sets of textual documents. Whether using machine learning or statistical techniques, the text mining approaches are usually language independent. However, specially in the natural language processing field, annotated corpora is often required to train models in order to resolve a certain task for each specific language (semantic role labeling problem is an example). Besides, linguistic resources as semantic networks or lexical databases, which are language-specific, can be used to enrich textual data. Thus, the low number of annotated data or linguistic resources can be a bottleneck when working with another language. Semantic analysis, in the broadest sense, is the process of interpreting the meaning of text.
What is the purpose of semantics?
The aim of semantics is to discover why meaning is more complex than simply the words formed in a sentence. Semantics will ask questions such as: “Why is the structure of a sentence important to the meaning of the sentence? “What are the semantic relationships between words and sentences?”
Sentiment analysis and semantic analysis are popular terms used in similar contexts, but are these terms similar? The paragraphs below will discuss this in detail, outlining several critical points. Data science involves using statistical and computational methods to analyze large datasets and extract insights from them. However, traditional statistical methods often fail to capture the richness and complexity of human language, which is why semantic analysis is becoming increasingly important in the field of data science. Despite the advancements in semantic analysis for LLMs, there are still several challenges that need to be addressed. Words and phrases can have multiple meanings depending on the context, making it difficult for machines to accurately interpret their meaning.
Unleashing the Potential of SAP Customer Experience Cloud: Transforming Customer Engagement
The goal of NER is to extract and label these named entities to better understand the structure and meaning of the text. This method involves generating multiple possible next words for a given input and choosing the one that results in the highest overall score. The training process also involves a technique known as backpropagation, which adjusts the weights of the neural network based on the errors it makes. This process helps the model to learn from its mistakes and improve its performance over time. Semantic analysis aids in analyzing and understanding customer queries, helping to provide more accurate and efficient support.
With the availability of NLP libraries and tools, performing sentiment analysis has become more accessible and efficient. As we have seen in this article, Python provides powerful libraries and techniques that enable us to perform sentiment analysis effectively. By leveraging these tools, we can extract valuable insights from text data and make data-driven decisions. One of the most common applications of semantics in data science is natural language processing (NLP). NLP is a field of study that focuses on the interaction between computers and human language. It involves using statistical and machine learning techniques to analyze and interpret large amounts of text data, such as social media posts, news articles, and customer reviews.
This understanding is crucial for the model to generate coherent and contextually relevant responses. Another crucial aspect of semantic analysis is understanding the relationships between words. Words in a sentence are not isolated entities; they interact with each other to form meaning. For instance, in the sentence “The cat chased the mouse”, the words “cat”, “chased”, and “mouse” are related in a specific way to convey a particular meaning. In the context of LLMs, semantic analysis is a critical component that enables these models to understand and generate human-like text. It is what allows models like ChatGPT to generate coherent and contextually relevant responses, making them appear more human-like in their interactions.
The dbt Semantic Layer provides the flexibility to define metrics on top of your existing models and then query those metrics and models in your analysis tools of choice. Now, we can understand that meaning representation shows how to put together the building blocks of semantic systems. In other words, it shows how to put together entities, concepts, relation and predicates to describe a situation. It is the first part of the semantic analysis in which the study of the meaning of individual words is performed.
How to build a semantic data model?
- Create an empty semantic model.
- Import an exported semantic model (. rpd file), an archived semantic model (. zip file), or an .
- Load the semantic model deployed to Oracle Analytics.
- Clone a Git repository to your development environment.
Both polysemy and homonymy words have the same syntax or spelling but the main difference between them is that in polysemy, the meanings of the words are related but in homonymy, the meanings of the words are not related. In the above sentence, the speaker is talking either about Lord Ram or about a person whose name is Ram. In-Text Classification, our aim is to label the text according to the insights we intend to gain from the textual data. Likewise, the word ‘rock’ may mean ‘a stone‘ or ‘a genre of music‘ – hence, the accurate meaning of the word is highly dependent upon its context and usage in the text.
Semantics is an essential component of data science, particularly in the field of natural language processing. Applications of semantic analysis in data science include sentiment analysis, topic modelling, and text summarization, among others. As the amount of text data continues to grow, the importance of semantic analysis in data science will only increase, making it an important area of research and development for the future of data-driven decision-making. Semantics is a branch of linguistics, which aims to investigate the meaning of language. Semantics deals with the meaning of sentences and words as fundamentals in the world.
“Single-concept perception”, “Two-concept perception”, “Entanglement measure of semantic connection” sections describe a model of subjective text perception and semantic relation between the resulting cognitive entities. Semantics gives a deeper understanding of the text in sources such as a blog post, comments Chat GPT in a forum, documents, group chat applications, chatbots, etc. With lexical semantics, the study of word meanings, semantic analysis provides a deeper understanding of unstructured text. Beside Slovenian language it is planned to be possible to use also with other languages and it is an open-source tool.
With the help of semantic analysis, machine learning tools can recognize a ticket either as a “Payment issue” or a“Shipping problem”. Now, we have a brief idea of meaning representation that shows how to put together the building blocks of semantic systems. In other words, it shows how to put together entities, concepts, relations, and predicates to describe a situation. With the rise in machine learning and artificial intelligence approaches to big data, systems that can integrate into the complex ecosystem typically found within large enterprises are increasingly important. This semantic enrichment opens up new possibilities for you to mine data more effectively, derive valuable insights and ensure you never miss something relevant.
For the further development and practical implications of the tool, it is important that the content and form of the texts and data collections which are used for searching, are complete, updated, and credible. An appropriate support should be encouraged and provided to collection custodians to equip them to align with the needs of a digital economy. Each collection needs a custodian and a procedure for maintaining the collection on a daily basis. What we have learned from ChatGPT is the self-serve capabilities and quick access to the correct information without a middle person is the key to win the market. If we want to nurture a self-serve analytics culture in our organizations, we need to make investment into our semantic layer.
It is a collection of procedures which is called by parser as and when required by grammar. Both syntax tree of previous phase and symbol table are used to check the consistency of the given code. Type checking is an important part of semantic analysis where compiler makes sure that each operator has matching operands. Continue reading this blog to learn more about semantic analysis and how it can work with examples. Hence, under Compositional Semantics Analysis, we try to understand how combinations of individual words form the meaning of the text.
For example, you might decide to create a strong knowledge base by identifying the most common customer inquiries. Using the tool increases efficiency when browsing through different sources that are currently unrelated. We would also like to emphasise that the search is performed among credible sources that contain reliable and relevant information, which is of paramount importance in today’s flood of information on the Internet. Latent Semantic Analysis (LSA) is a theory and method for extracting and representing the contextual-usage meaning of words by statistical computations applied to a large corpus of text. Right
now, sentiment analytics is an emerging
trend in the business domain, and it can be used by businesses of all types and
sizes. Even if the concept is still within its infancy stage, it has
established its worthiness in boosting business analysis methodologies.
It’s not just about understanding text; it’s about inferring intent, unraveling emotions, and enabling machines to interpret human communication with remarkable accuracy and depth. From optimizing data-driven strategies to refining automated processes, semantic analysis serves as the backbone, transforming how machines comprehend language and enhancing human-technology interactions. Businesses need a tool that can create abstractions of mountains of data from disparate sources, contextualize it, and glean actionable insights for data-driven decisions – and they need a tool that can do that every day. How can enterprises prepare for these rapidly approaching (and growing) needs for handling future data workloads?
- It is a powerful application of semantic analysis that allows us to gauge the overall sentiment of a given piece of text.
- This tool has significantly supported human efforts to fight against hate speech on the Internet.
- In the dynamic landscape of customer service, staying ahead of the curve is not just a… To classify sentiment, we remove neutral score 3, then group score 4 and 5 to positive (1), and score 1 and 2 to negative (0).
- SciBite uses semantic analytics to transform the free text within patient forums into unambiguous, machine-readable data.
The process
involves various creative aspects and helps an organization to explore aspects
that are usually impossible to extrude through manual analytical methods. The
process is the most significant step towards handling and processing
unstructured business data. Consequently, organizations can utilize the data
resources that result from this process to gain the best insight into market
conditions and customer behavior. QuestionPro often includes text analytics features that perform sentiment analysis on open-ended survey responses. While not a full-fledged semantic analysis tool, it can help understand the general sentiment (positive, negative, neutral) expressed within the text.
Qualitative Intelligence Debuts Predictive Analytics for Real-Time Message Testing and Risk Assessment, With NEC’s … – Yahoo Finance
Qualitative Intelligence Debuts Predictive Analytics for Real-Time Message Testing and Risk Assessment, With NEC’s ….
Posted: Wed, 12 Jun 2024 15:00:00 GMT [source]
Semantic analysis, also known as semantic processing or semantic understanding, is a field within natural language processing (NLP) that focuses on understanding the meaning and context from natural language text or speech. It involves analyzing the relationships between words, identifying concepts, and understanding the overall intent or sentiment expressed in the text. Semantic analysis goes beyond simple keyword matching and aims to comprehend the deeper meaning and nuances of the language used.
Besides the vector space model, there are text representations based on networks (or graphs), which can make use of some text semantic features. Network-based representations, such as bipartite networks and co-occurrence networks, can represent relationships between terms or between documents, which is not possible through the vector space model [147, 156–158]. This technique is used separately or can be used along with one of the above methods to gain more valuable insights. With the help of meaning representation, we can link linguistic elements to non-linguistic elements.
Analyzing the provided sentence, the most suitable interpretation of “ring” is a piece of jewelry worn on the finger. Now, let’s examine the output of the aforementioned code to verify if it correctly identified the intended meaning. One approach to improve common sense reasoning in LLMs is through the use of knowledge graphs, which provide structured information about the world. Another approach is through the use of reinforcement learning, which allows the model to learn from its mistakes and improve its performance over time. Semantic analysis, the engine behind these advancements, dives into the meaning embedded in the text, unraveling emotional nuances and intended messages.
Google’s Hummingbird algorithm, made in 2013, makes search results more relevant by looking at what people are looking for. Semantic Analysis is a topic of NLP which is explained on the GeeksforGeeks blog. The entities involved in this text, along with their relationships, are shown below. Semantic analysis also takes into account signs and symbols (semiotics) and collocations (words that often go together).
In accord, this makes a powerful navigator in space of behavioral and linguistic models as discussed in more detail in “Discussion” section. A detailed literature review, as the review of Wimalasuriya and Dou [17] (described in “Surveys” section), would be worthy for organization and summarization of these specific research subjects. The second most used source is Wikipedia [73], which covers a wide range of subjects and has the advantage of presenting the same concept in different languages. Wikipedia concepts, as well as their links and categories, are also useful for enriching text representation [74–77] or classifying documents [78–80].
By building with the right kind of universal semantic layer – one that opens the gates for data literacy for any and all users. The semantic layer platform is integrated into the consumption platform — the analytics tools such as Power BI, Tableau, Python, Business Objects, Looker, Jupyter Notebook, and even Microsoft Excel. The queries from the business users could be in SQL, DAX, MDX, and so on using the tool-specific native protocols such as XMLA, JDBC, ODBC, SOAP, and REST interfaces. By abstracting the physical form and location of data, the semantic layer platform makes data stored in the data warehouse, data lake, or data mart accessible with one consistent and secure interface for business users.
This data is used to train the model to understand the nuances and complexities of human language. The training process involves adjusting the weights of the neural network based on the errors it makes in predicting the next word in a sentence. Over time, the model learns to generate more accurate predictions, thereby improving its understanding of language semantics.
It achieves this by mapping the business terms and concepts that users are familiar with to the corresponding data elements in the data sources. Today, the word “semantic” has become an integral part of various academic and technical domains, enriching our understanding of communication, cognition, and the intricacies of human language. In its simplest form, semantic analysis is the process that extracts meaning from text. Applying semantic analysis in natural language processing can bring many benefits to your business, regardless of its size or industry. If you wonder if it is the right solution for you, this article may come in handy.
Semantic analytics measures the relatedness of different ontological concepts. Automated semantic analysis works with the help of machine learning algorithms. It’s an essential sub-task of Natural Language Processing (NLP) and the driving force behind machine learning tools like chatbots, search engines, and text analysis.
Very close to lexical analysis (which studies words), it is, however, more complete. This improvement of common sense reasoning can be achieved through the use of reinforcement learning, which allows the model to learn from its mistakes and improve its performance over time. It can also be achieved through the use of external databases, which provide additional information that the model can use to generate more accurate responses. LLMs like ChatGPT use a method known as context window to understand the context of a conversation. The context window includes the recent parts of the conversation, which the model uses to generate a relevant response. This understanding of context is crucial for the model to generate human-like responses.
In LLMs, this understanding of relationships between words is achieved through vector representations of words, also known as word embeddings. These embeddings capture the semantic relationships between words, enabling the model to understand the meaning of sentences. Semantic analysis significantly improves language understanding, enabling machines to process, analyze, and generate text with greater accuracy and context sensitivity. Besides that, users are also requested to manually annotate or provide a few labeled data [166, 167] or generate of hand-crafted rules [168, 169].
What are the benefits of semantic data model?
Benefits of Semantic Data Modeling
Semantic models provide a common, shared understanding of data across different systems, applications, and domains. This semantic interoperability enables seamless data integration, reducing the need for complex mappings and transformations.
Improved conversion rates, better knowledge of the market… The virtues of the semantic analysis of qualitative studies are numerous. Used wisely, it makes it possible to segment customers into several targets and to understand their psychology. The study of their verbatims allows you to be connected to their needs, motivations and pain points. Research on the user experience (UX) consists of studying the needs and uses of a target population towards a product or service.
A semantic layer maps business data into familiar business terms to offer a unified, consolidated view of data across the organization and meet the growing analytics needs of an enterprise. The semantic layer manages the relationships between the various data attributes to create a simple and unified business view that can be used for querying and deriving insights quickly and cost-effectively. In this discussion, we are focused on semantic layers for analytics use cases — i.e. The term semantic layer is sometimes also used to describe knowledge graphs that support data exploration in large complex data sets.
You can foun additiona information about ai customer service and artificial intelligence and NLP. Using semantic analysis in the context of a UX study, therefore, consists in extracting the meaning of the corpus of the survey. Improvement of common sense reasoning in LLMs is another promising area of future research. This involves training the model to understand the world beyond the text it is trained on. For instance, understanding that a person cannot be in two places at the same time, or that a person needs to eat to survive. However, many organizations struggle to capitalize on it because of their inability to analyze unstructured data.
Democratizing data and generating insights have never been more important to achieving a competitive advantage. On the one hand, that means there’s more data available for analysis and reporting – which is great news. On the other hand, that means accurate, efficient analysis of incoming data requires more resources and firepower than ever – which can be a strain. The solution lies in having one standard and consistent definition for this business entity where “prospect,” “client,” and “counterpart” are mapped to one data entity. With the semantic layer, different data definitions from different sources can be quickly mapped for a unified and single view of data.
The word is assigned a vector that reflects its average meaning over the training corpus. Based on them, the classification model can learn to generalise the classification to words that have not previously occurred in the training set. Thibault is fascinated by the power of UX, especially user research and nowadays the UX for Good principles. As an entrepreneur, he’s a huge fan of liberated company principles, where teammates give the best through creativity without constraints.
Beyond just understanding words, it deciphers complex customer inquiries, unraveling the intent behind user searches and guiding customer service teams towards more effective responses. A typical feature extraction application of Explicit Semantic Analysis (ESA) is to identify the most relevant features of a given input and score their relevance. Therefore, in semantic analysis with machine learning, computers use Word Sense Disambiguation to determine which meaning is correct in the given context. The assignment of meaning to terms is based on what other words usually occur in their close vicinity. To create such representations, you need many texts as training data, usually Wikipedia articles, books and websites. Semantic
and sentiment analysis should ideally combine to produce the most desired outcome.
We utilize specific AI components and capabilities precisely when, where, and how you need them. We will calculate the Chi square scores for all the features and visualize the top 20, here terms or words or N-grams are features, and positive and negative are two classes. Given a feature X, we can use Chi square test to evaluate its importance to distinguish the class. I will show you how straightforward it is to conduct Chi square test based feature selection on our large scale data set. In reference to the above sentence, we can check out tf-idf scores for a few words within this sentence. The Metric Layer refers to a set of predefined metrics and key performance indicators (KPIs) that are essential for tracking and measuring specific business goals or objectives.
You can foun additiona information about ai customer service and artificial intelligence and NLP. To learn more and launch your own customer self-service project, get in touch with our experts today. The most important task of semantic analysis is to get the proper meaning of the sentence.
For example, if we talk about the same word “Bank”, we can write the meaning ‘a financial institution’ or ‘a river bank’. In that case it would be the example of homonym because the meanings are unrelated to each other. The primary role of Resource Description Framework (RDF) is to store meaning with data and represent it in a structured way that is meaningful to computers. https://chat.openai.com/ Mark contributions as unhelpful if you find them irrelevant or not valuable to the article. Understanding the sentiments of the content can help determine whether it’s suitable for certain types of ads. For instance, positive content might be suitable for promoting luxury products, while negative content might not be appropriate for certain ad campaigns.
What is the meaning of NLP?
Natural language processing (NLP) is a machine learning technology that gives computers the ability to interpret, manipulate, and comprehend human language.
What does a semantic analyzer do?
What is Semantic Analysis? Semantic analysis is the task of ensuring that the declarations and statements of a program are semantically correct, i.e, that their meaning is clear and consistent with the way in which control structures and data types are supposed to be used.
What is analytical approach in semantics?
Semantic analysis is the process of interpreting and understanding the meaning of words, phrases, and sentences within a language. It involves examining the relationship between words and their meanings in context, as well as identifying variations, ambiguities, and possible interpretations.
Top 5 Programming Languages For Artificial Intelligence
With libraries like OpenCV and sci-kit-image, Python enables developers to build applications that can recognize faces and objects, and even interpret complex scenes. From security systems to augmented reality, Python’s role in computer vision is indispensable. A vast and active Python community continually contributes to AI development. Abundant online resources, tutorials, and forums assist developers at all skill levels. But one of Haskell’s most interesting features is that it is a lazy programming language. Julia’s wide range of quintessential features also includes direct support for C functions, a dynamic type system, and parallel and distributed computing.
Packages such as `caret`, `randomForest`, and `boost` empower developers to implement a wide range of machine learning algorithms, from classification and regression to clustering. In summary, the best language to learn for machine learning and AI is the one that aligns with your project requirements, skill set, and personal or organizational goals. Each language covered above has its unique strengths and is best suited to particular types of tasks within the AI and ML landscapes. Where Python is interpreter-based, Julia uses a JIT (just in time) compilation – allowing it to yield faster execution. Similar to JavaScript, it is a dynamically typed programming language and has a built-in package manager and superior syntax compared to C and C++.
AI Programming With Julia
The field of AI is rapidly evolving, and Engineers like you must be equipped with the right tools to keep up. General-purpose programming languages like Python are great for getting started with Data Science and building Machine Learning models. It is popular for its outstanding prototyping capabilities as well as the simple dynamic creation of new objects, along with automatic garbage collection.
Python, the most popular and fastest-growing programming language, is an adaptable, versatile, and flexible language with readable syntax and a vast community. Many programming languages are commonly used for AI, but there is a handful that are not suitable for it. Perl is one example of a programming language that is typically not used for AI because it is a scripting language.
It is not as efficient or fast as languages like C++ or Python, and it was not designed with mathematical computations in mind, which are core to AI and ML algorithms. Also, while it is improving, JavaScript’s library ecosystem for AI and ML is not as mature as that of Python. Its learning curve is a little steep, especially for those not familiar with functional programming paradigms. Also, while its community is active, it’s not as large or as resource-rich as Python’s or Java’s.
Processing power acts as the driving force for AI, and your chosen programming language should seamlessly leverage this power. It’s akin to having a high-performance vehicle navigating through traffic effortlessly. Seek a language that adeptly manages extensive datasets and easily adapts to new hardware upgrades without requiring a complete code overhaul. When it comes to performance-intensive AI and ML applications, C++ might very well be the AI best programming language. Its unmatched control over system resources and memory management makes C++ the go-to language for situations where speed and efficiency are non-negotiable, such as in real-time systems and robotics.
Prolog is also used for natural language processing and knowledge representation. Lisp stands out for AI systems built around complex symbolic knowledge or logic, like automated reasoning, natural language processing, game-playing algorithms, and logic programming. It represents information naturally as code and data symbols, intuitively encoding concepts and rules that drive AI applications.
Julia can execute numerical and scientific computing tasks quickly and efficiently. After Python, R is another favored language among statisticians and AI and machine learning practitioners. Due to its specialized focus, R has a distinctive place in the programming language world. Python is essential to programming, especially when discussing AI and machine learning. Look at the section below to learn what makes Python a preferred choice and explore its strengths. AI and machine learning allow businesses to securely and efficiently store, process, and analyze large datasets from various sources.
Python’s readability and ease of use make it an excellent choice for beginners in AI development. It has a large community of developers who contribute to open-source libraries and tools, making it easy to find solutions to common problems. Java is a universally useful programming language that is generally utilized in big business applications. It has a rich environment of libraries and systems for simulated AI development, like Deeplearning4j and Weka. In recent years, Artificial Intelligence has seen exponential growth and innovation in the field of technology.
It can be worth considering specializing in a sub-field aligning with personal interests like natural language processing, computer vision, or robotics, Singh Ahuja says. Prioritizing ethics and understanding the true implications of AI are best programming language for ai also critical. Regardless, having foundation skills in a language like Python can only help you in the long run. Enrolling in a Python bootcamp or taking a free online Python course is one of many ways to learn the skills to succeed.
Furthermore, the heavy use of parentheses in Lisp can be off-putting to those accustomed to C-like syntax. While it does not offer the same kind of library support as Python, Lisp has always been popular in academia and artificial intelligence research. It was, after all, created as a practical mathematical notation for computer programs. This mathematical foundation is particularly handy when implementing complex machine-learning algorithms. Julia’s ability to execute numerical and scientific computing tasks quickly and efficiently makes it a potent tool for AI and machine learning.
With a passion for technology and an immaculate drive for entrepreneurship, Harnil has propelled Hyperlink InfoSystem to become a global pioneer in the world of innovative IT solutions. His exceptional leadership has inspired a multiverse of tech enthusiasts and also enabled thriving business expansion. Outside his duties at Hyperlink InfoSystem, Harnil has earned a reputation for his conceptual leadership and initiatives in the tech industry. He is driven to impart expertise and insights to the forthcoming cohort of tech innovators.
Python or R? Choosing the Right Tool for Your Data Science Journey
Furthermore, Haskell’s ecosystem for AI and machine learning, though growing, is not as extensive or mature as those of more commonly used languages. For instance, MLpack is a machine learning library in C++ that emphasizes speed and flexibility. Libraries like Dlib, known for their real-time processing capabilities, and Tensorflow, which has C++ API in addition to its primary Python interface, are also available for use. It has a steeper learning curve than other languages like Python and R, which can deter beginners. While powerful, its syntax is more complex and less readable, requiring a solid understanding of programming concepts.
There are several that can serve to make your AI integration dreams come true. Let’s dive in and take a look at 9 of the best languages available for Artificial Intelligence. Java’s position as a major player in mobile application development converges seamlessly with the evolving AI landscape. Armed with libraries such as the Deep Java Library, Kubeflow, OpenNLP, and the Java Machine Learning Library, Java is proving to be a solid foundation for seamless AI implementation. And as it’s transforming the way we live and is changing the way we interact with the world and each other, it’s also creating new opportunities for businesses and individuals. These are languages that, while they may have their place, don’t really have much to offer the world of AI.
The language’s efficiency ensures real-time processing of audio data, a fundamental requirement in speech recognition. C++ stands as a stalwart in the world of programming languages, and its relevance in AI is far from fading. Here’s a closer look at why C++ is not just holding its ground but thriving in the field of Artificial Intelligence. Java’s object-oriented approach facilitates the development of robust and modular code for robotics applications.
C++, a programming language with a storied history, remains a formidable contender in AI. Despite the emergence of newer languages, C++ continues to hold its ground. Java’s intersection with AI programming creates a powerful synergy, amplifying the capabilities of AI in the mobile app landscape.
Java’s object-oriented nature not only aligns with the demands of AI development but also provides a robust foundation for creating sophisticated and scalable AI applications. Its symbolic processing strength finds application in expert systems, where logical reasoning and decision-making are crucial components. Natural Language Processing (NLP) applications, from chatbots to sentiment https://chat.openai.com/ analysis, leverage Python’s elegance. Libraries like NLTK and spaCy make handling text a breeze, allowing developers to delve into the intricacies of language effortlessly. Python’s simplicity and readability make it one of the easiest languages for beginners to learn and use. Its syntax, resembling pseudo-code, promotes a straightforward and intuitive approach to programming.
Java, on the other hand, is a versatile language with scalability and integration capabilities, making it a preferred choice in enterprise environments. JavaScript, the most popular language for web development, is also used in web-based AI applications, chatbots, and data visualization. R stands out for its ability to handle complex statistical analysis tasks with ease. It provides a vast ecosystem of libraries and packages tailored specifically for statistical modeling, hypothesis testing, regression analysis, and data exploration.
Furthermore, Perl’s syntax can be challenging to grasp for beginners, making it less approachable for AI and machine learning tasks. While Lisp might not be the first language that comes to mind for modern AI and ML applications, it has left an indelible mark on the history of AI programming. It might not be the go-to choice for most developers today, but its legacy continues to influence many modern programming languages, and it remains an interesting option for certain AI-focused projects. Its popularity and usage have significantly diminished over the years, resulting in smaller community support. The availability of resources, tools, libraries, and tutorials is rather limited when compared to languages like Python or Java.
A vibrant and active developer community contributes to a wealth of resources, tutorials, and frameworks for integrating AI into JavaScript-based projects. Julia’s built-in capabilities for parallel and distributed computing are particularly advantageous in AI applications that demand extensive computational power. While R may not be the first choice for image processing, it has capabilities through packages like `EBImage` and `imager`. These packages allow for basic image analysis and processing, making R a viable option for certain AI applications involving images. R plays a role in NLP applications, thanks to packages like `tm` (Text Mining) and `NLP`.
A good example is TensorFlow.js, which runs directly within the browser and opens up many possibilities for web developers. Building your knowledge of browser-based AI applications can help you build next-generation AI-focused browser tools. Java is an incredibly powerful language used across many software development contexts. It’s especially prevalent in the mobile app space, where many applications are taking advantage of artificial intelligence features. Python’s rise is due in large part to its robust data analysis capabilities, which are complemented by specialized AI frameworks such as TensorFlow, scikit-learn, PyTorch, Keras, and Theano.
Python has potentially established its position as a data analysis tool and is heavily leveraged in the big data space. Being one of the simplest programming languages to learn and implement, Python constantly ranks as the top AI-specific framework. It also has a humongous ecosystem of frameworks and libraries such as Matplotlib, Scikit-learn, and NumPy in addition to its Python-based frameworks PyTorch and TensorFlow. This versatile programming language is primarily used to develop neural networks and algorithms in machine learning. Python is also useful for analyzing data in order to uncover patterns, behaviors, anomalies, potential trends, and other relationships due to its open-source nature.
From conceptualization to deployment, we pledge to bestow inventive and dependable software solutions that empower you to maintain an edge in the competitive landscape. Cons- C++ gives developers manual command over the memory of the executives, which can be both a benefit and a test. While it takes into consideration fine-grained control and performance optimization, improper memory handling can prompt bugs, memory leaks, or indistinct ways of behaving. Remember, choosing a language isn’t about picking the ‘best’ one – it’s about picking the right one for you and your project. Whether you’re dancing at a black-tie event or chilling on a sandy beach, make sure you have the right shoes… or, in our case, the right language. Java’s platform independence, captured in the phrase “Write Once, Run Anywhere,” makes it highly portable.
10 Best AI Code Generators (June 2024) – Unite.AI
10 Best AI Code Generators (June .
Posted: Sun, 02 Jun 2024 07:00:00 GMT [source]
Libraries like MapReduce facilitate distributed computing, mlpack excels in machine learning tasks, and MongoDB leverages C++ for efficient data management in AI applications. C++ is another language that has been around for quite some time, but still is a legitimate contender for AI use. One of the reasons for this is how widely flexible the language is, which makes it perfectly suited for resource-intensive applications. C++ is a low-level language that provides better handling for the AI model in production.
Beyond Coding: AI Copilots Are Taking the Lead in the Workplace
Python has been used to build a number of AI systems, as it is highly intuitive, which makes it easy to understand for scientists and non-programmers alike. But you’ll need capable software developers if you want to integrate artificial intelligence into your business systems and services. You can foun additiona information about ai customer service and artificial intelligence and NLP. Furthermore, such programmers will need to get acquainted with the optimal languages that could be used to develop AI. Overall, Python’s versatility and ease of use make it an excellent choice for a wide range of AI applications, from natural language processing and computer vision to robotics and game development. By understanding the strengths and features of each language, developers can choose the best tools to drive their AI projects to success.
Julia is a prominent framework among the AI community, and one of the programming languages for AI development due to its elevated performance and swift prototyping capabilities. Its faster processing abilities are crucial for AI development due to the involvement of huge amounts of data. Its user-friendly syntax is analogous to Python, making it more straightforward to understand. Julia is highly compatible with prominent programming languages like Python, R, and C. This enables developers to utilize powerful libraries and dependencies such as PyTorch and TensorFlow, that are typed in other languages, and further integrate them into their applications.
TensorFlow and PyTorch, for instance, have revolutionized the way AI projects are built and deployed. These frameworks simplify AI development, enable rapid prototyping, and provide access to a wealth of pre-trained models that developers can leverage to accelerate their AI projects. The programming language supports fundamental mechanisms like tree-based data structuring, pattern matching, and automatic backtracking necessary for the purpose of AI programming. In addition to its wide use into different AI projects, it is known that Prolog is used for the preparation of medical systems. The language is capable to compete another programming language –Lisp for AI programming.
Today, Lisp is used in a variety of applications, including scripting and system administration. Scala thus combines advanced language capabilities for productivity with access to an extensive technology stack. Java is used in AI systems that need to integrate with existing business systems and runtimes. The language boasts a range of AI-specific libraries and frameworks like scikit-learn, TensorFlow, and PyTorch, covering core machine learning, deep learning, and high-level neural network APIs.
It comes with many libraries that can be leveraged to create neural networks and preprocess data tasks. Yes, R can be used for AI programming, especially in the field of data analysis and statistics. R has a rich ecosystem of packages for statistical analysis, machine learning, and data visualization, making it a great choice for AI projects that involve heavy data analysis.
Python is emerged as one of the fastest-adopted languages for Artificial intelligence due to its extensive libraries and large community support. Also, to handle the evolving challenges in the Artificial intelligence field, you need to stay updated with the advancements in AI. Nevertheless, various notable JavaScript libraries are available for AI and ML. TensorFlow.js, a JavaScript library for training and deploying machine learning models in the browser and on Node.js, stands out prominently. For natural language processing, there’s compromise, and for machine learning, there’s machinelearn.js, amongst others.
- Haskell is a functional programming language that focuses on precise mathematical computation for AI algorithms.
- With tools like Apache Spark and Hadoop, you can process and analyze enormous datasets across clusters of computers.
- Apart from rendering superior AI model management, it also helps in building flawless learning libraries for deep learning and machine learning.
- It is also capable of meeting multiple requirements and working in various environments.
JavaScript’s versatility makes it an excellent choice for AI tasks in web development. It seamlessly integrates with HTML and CSS, facilitating the creation of dynamic and interactive user interfaces for AI-powered applications. The declarative nature of Prolog allows programmers to represent knowledge concisely and logically. This makes it valuable for applications requiring the representation of complex relationships, making it easier to express and manipulate knowledge. The language utilizes a tree-based data structuring approach, facilitating the representation and manipulation of hierarchical structures.
R language:
However, Python has its downsides when it comes to AI programming despite the vast ecosystem and use cases, due to performance limitations. Consequently, it is often avoided in applications requiring quick responses. As a result, several large-scale AI projects prefer lower-level languages for better performance. This article explores the Top 10 AI programming languages commonly used for Development projects. Bring your unique software vision to life with Flatirons’ custom software development services, offering tailored solutions that fit your specific business requirements.
5 Best Open Source LLMs (June 2024) – Unite.AI
5 Best Open Source LLMs (June .
Posted: Mon, 03 Jun 2024 07:00:00 GMT [source]
This visual representation aids in comprehending complex AI models and results, enhancing the overall understanding of the system. Lisp excels in symbolic information processing, a key aspect in AI applications where the interpretation of symbols and meanings is paramount. Lisp facilitates the swift development of prototypes, a critical factor in the dynamic and evolving field of artificial intelligence.
Other things that can accelerate the development process include rich ecosystem of tools, libraries and the right framework. Selecting the right programming language for AI development depends on the your project objectives. Python stands out for its versatility, short development time and extensive library support, making it an excellent choice for Chat GPT many AI applications. Java offers reliability and scalability, suitable for enterprise-level AI solutions. R excels in statistical analysis and data visualization, while Julia provides high performance for computational-heavy tasks. Finally, C++ is unmatched in performance and control, ideal for real-time and resource-intensive AI applications.
There are a bunch of options out there, and it can be overwhelming to figure out which one will be the best fit for your projects. In this guide, we’ll explore the top programming languages for data science and AI and help you make a decision based on your needs and the tools available. Scala’s expressive syntax and support for functional programming concepts are beneficial in developing NLP applications. Combining Scala with libraries like Apache OpenNLP allows developers to create sophisticated natural language processing solutions.
The development cycle of Lisp is such that it permits interactive assessment of expressions as well as recompilation of file or functions when the program is still executing. As the time passed, several of its features have transferred into several other programming languages thus influencing Lisp’s uniqueness. For the development of software applications, a developer has many different options to choose among the languages for writing the programs. Similar to that for AI development, there are lots of programming languages to choose from. The choice of programming language and the development process relies on the anticipated functionality of the AI application to be developed. Historically, some programming languages have been specifically designed for artificial intelligence (AI) applications.
This implies there might be fewer libraries and devices accessible for specific tasks, and finding support or resources can sometimes be more challenging. In 2024, organizations are wholeheartedly embracing cutting-edge AI technologies to maximize efficiency and foster innovation. By integrating AI into workflows, they aim to unlock unprecedented productivity and competitive advantage.
Its major focus is on formal logic, which is perfect for rule-based AI systems. Many languages can work well with AI, but there are some that programmers, especially ChatGPT, shouldn’t use. Here is a list of some programming languages unsuitable for AI and machine learning.
These types of tools allow interfaces and graphics appear attractive and classy. You will find that the coding of algorithms is easy and it encompasses a key part of AI. In this blog, we delve into the five popular programming languages for AI development, each with its unique strengths and capabilities. Prolog is an excellent choice for AI projects involving logic and symbolic reasoning, thanks to its inherent support for pattern matching and automatic backtracking. It excels in domains such as rule-based systems, expert systems, and natural language processing.
Developed by Facebook’s AI Research lab, PyTorch is another popular library for machine learning that is especially well-suited for applications like natural language processing. It is known for its simplicity and ease of use, especially when it comes to building deep learning models. Developed by Google, TensorFlow is a leading library for creating and training machine learning models, including deep learning models.
- More importantly, the man who created Lisp (John McCarthy) was very influential in the field of AI, so much of his work had been implemented for a long time.
- It works well with other AI programming languages, but has a steep learning curve.
- Java’s position as a major player in mobile application development converges seamlessly with the evolving AI landscape.
- This integration signifies a leap toward a future of intelligent automation, optimizing processes, and nurturing revolutionary solutions.
- Staying knowledgeable about cutting-edge AI programming languages allows developers to stay competitive and deliver innovative AI solutions.
Ultimately, the best AI language for you is the one that is easiest for you to learn. Other top contenders include Java, C++, and JavaScript — but Python is likely the best all-around option for AI development. For example, in the case of machine learning, you might choose Python, which is a great choice for this AI subset. If your company requires the addition of Artificial Intelligence development services, you need to begin the process of integrating one or more of these languages.
The examples page showcases many implementations of the library, from training a neural network to remember a string of characters, to deciphering captchas. The library shows the depth of what you can achieve when using Java for AI development. Python is very adaptable and can be used for many machine learning and AI-focused applications — you can find a repository of practical AI-focused projects on GitHub. If you’re interested in learning more about web development languages that can be applied in artificial intelligence, consider signing up for Berkeley Coding Boot Camp.
Raised in Buenos Aires, Argentina, he’s a musician who loves languages (those you use to talk to people) and dancing. While Python is still preferred across the board, both Java and C++ can have an edge in some use cases and scenarios. For example, C++ could be used to code high-performance routines, and Java could be used for more production-grade software development. As with everything in IT, there’s no magic bullet or one-size-fits-all solution. C++ has also been found useful in widespread domains such as computer graphics, image processing, and scientific computing. Similarly, C# has been used to develop 3D and 2D games, as well as industrial applications.